SYDNEY J. FREEDBERG JR.
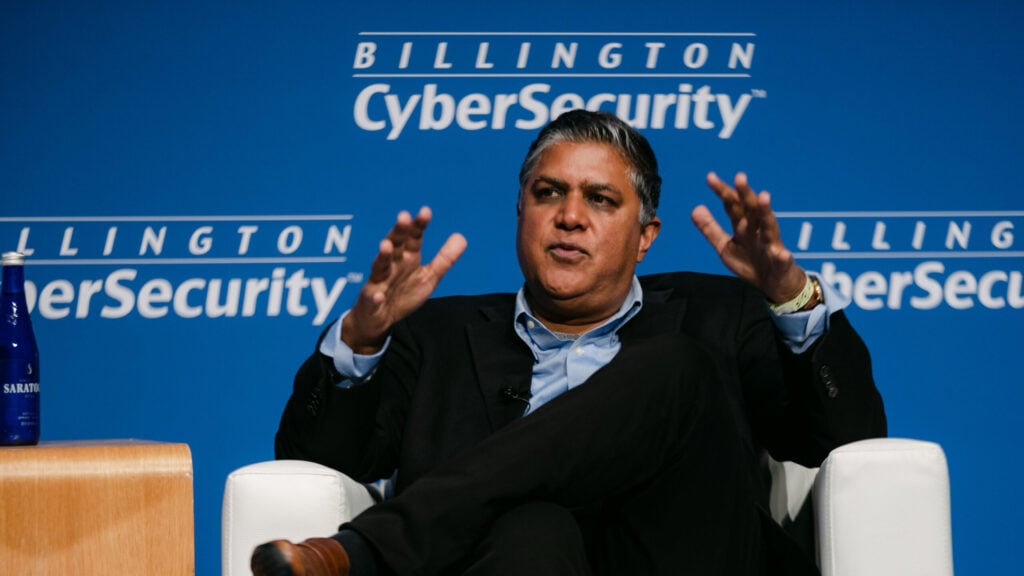
WASHINGTON — Can intelligence agencies trust artificial intelligence? From ChatGPT’s plausible but erroneous answers to factual questions, to Stable Diffusion’s photorealistic human hands with way too many fingers, to some facial recognition algorithms’ inability to tell Black people apart, the answer is looking like “hell no.”
But that doesn’t mean the government shouldn’t use it, as long as officials take its insights and outputs with a healthy grain of salt. And even when it’s wrong, the CIA’s chief technology officer said this week, in some situations AI can be useful if its answers — regarded with appropriate suspicion — do nothing more than force analysts to examine the problem a different way and push them out of what he called “conceptual blindness.”
In those cases, treat AI, he said, not as an infallible oracle but “what I call the crazy drunk friend.”
AI “can give you something so far outside of your range, that it really then opens up the vista in terms of where you’re going to go,” said Nand Mulchandani, the Agency’s first-ever CTO (and former acting chief of the Pentagon’s Joint AI Center), at the Billington Cybersecurity Summit on Wednesday. “[This], I think, is actually the really interesting creative side of things.”
Mulchandani said AI-based systems are “absolutely fantastic… where you’re looking through large amounts of data, where you’re looking for patterns.” That’s why the CIA and other intelligence agencies, drowning in data, are so interested. “The intelligence [analysis] problems actually are incredibly well suited for the types of stuff that AI does very well.”
“But in areas where it requires precision,” he warned, “we’re going to be incredibly challenged.”
That’s because most widely-used algorithms work by looking for statistical correlations — for what’s probable, not what’s certain. (By contrast, an earlier generation of AIs, called expert systems, started with preprogrammed axioms held to be absolutely true — an approach that DARPA and others are reviving).
So if someone asks ChatGPT or similar Large Language Models about a factual question, for instance, the model doesn’t actually “know” any facts: It just calculates what word or word fragment (the technical term is “token”) is most likely to come next, based on scouring countless web pages.
Such “generative” AIs, which output original text or images, are particularly vulnerable to errors, known as “hallucinations,” but all machine learning systems can make spurious connections and draw false conclusions. What’s more, unlike traditional “if-then” “heuristic” software, machine learning doesn’t give the same output every time — again, it’s working with probabilities, not certainties — so its outputs can be profoundly unpredictable.
But it’s that unpredictability that can serve as a shock to the system. People who’ve spent years becoming knowledgeable about a specific subject always have limits to their knowledge, and the more specialized they are, the starker the difference between their area of expertise and everything outside it. The result, Mulchandani said, is such “domain experts” can suffer from “conceptual blindness… something so far outside of your purview or training, that you’re not really aware of.”
That’s one reason Mulchandani said he thinks the “current debate” over AI “los[es] sight of the fact that AI-based system, logic-based systems… offer very different benefits for different types of problems,” he said. “This idea of equally applying AI secularly to everything” – treating it like “peanut butter,” he said elsewhere – “is just not going to work.”
No comments:
Post a Comment