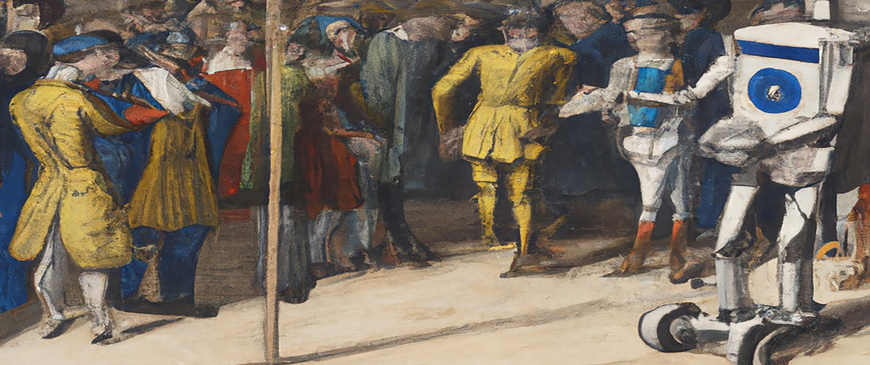
Artificial intelligence (AI) – computing systems that use a significant degree of autonomy and adaptability to achieve particular goals – is already being exploited by leading technology firms. But recent advances like ChatGPT have raised hopes that the technology could boost Europe’s slow-growing economy. Tech optimists argue AI will raise productivity growth in some services industries by doing (often boring) work in areas like research, accounting, contract and report drafting, coding and communication with customers. There are also hopes AI might help companies perform drug discovery, supply chain management, cancer screening and other technical tasks more cheaply, quickly, and accurately.
However, European politicians worry about the technology just as they did about previous digital innovations. In her recent State of the Union speech, European Commission President von der Leyen talked up the “risk of extinction from AI”. This alarmism is unwarranted for a technology that is useful, but currently no more than an advanced form of autocomplete. Another big fear is that AI will make Europe even more dependent on a few US tech giants. Yet others are concerned (with reason) about the risks of workers losing their jobs or compromising consumer safety. AI might replicate and amplify human biases against women and minorities, for example, help trouble-makers propagate misinformation, interfere in elections, pose cybersecurity risks or raise income inequality further. There are European and global efforts to deal with the safety risks that AI poses, but this paper limits itself to the potential effects of AI on economic activity and how Europe should seek to extract the most benefit from the technology.3
It is not surprising that the US, with its tech firms, skilled computer scientists and deep capital markets, became the global centre for the development of AI ‘foundation models’. These are the core AI models used for the analysis and processing of language, images and statistics, which firms will then adapt and deploy commercially. However, Europe would be wrong to hold back AI adoption on this basis: it is too early to conclude that a few technology firms will dominate markets in AI foundation models in the same way that they dominate more established digital markets like search engines.
And, in the meantime, the fine-tuning and adoption of AI by companies in the wider economy will take time, if the past is any guide. In 2021, only 8 per cent of EU enterprises used any form of AI.4 Speeding up the process of adoption in Europe should be policy-makers’ main focus. That will require incentives to adopt the technology, skilled workers to develop and adapt it, and new regulations to ensure AI is trustworthy and to provide certainty about the legal consequences of its use. By speeding up the take-up of AI, we will more quickly discover whether it will lead to sizeable productivity gains and help mitigate the risk of the EU’s productivity falling further behind.
WHAT IS ARTIFICIAL INTELLIGENCE?
AI does not have a single definition, but there is widespread agreement that AI refers to computing systems that can use a significant degree of autonomy and adaptability to achieve particular goals. Some elements of AI, such as automated decision-making and advanced data analysis, have been used for years and are already integrated into online products and software.
The example of how Google’s web search has evolved to incorporate AI illustrates how AI works. Google’s search engine was first created by humans coding algorithms. The algorithms promoted web pages that users were expected to find most useful, according to a combination of factors such as the search terms used, how much traffic the web page had, whether that web page was linked to by others, and user location, among other things. Now, however, AI can provide much more sophisticated results. For example, for years Google has used RankBrain, a machine learning algorithm, which can analyse the relationship between different words in a user’s search, to better understand the user’s intent. AI can also examine the context of the user’s query to identify typos. And it can examine the relevance of individual passages in webpages, rather than only looking at the webpage as a whole, to help identify the best result.5
This is just one example of AI’s many applications. What is unique to any AI application is its ability to discern statistical patterns in datasets without a human determining exactly how the AI should process the information it is given. This allows AI to find patterns too complex or time-consuming for a human to identify, and extrapolate from those patterns to make predictions. Large language models (LLMs), like ChatGPT, use statistical methods to produce text which the AI determines is most likely to be an appropriate response to a particular human query. Other forms of AI can perform tasks like recognising objects in images or processing human speech, powering semi-autonomous robots, and supporting decision-making. Some forms of AI can go further and continuously improve their own performance as they encounter new data or observe how humans respond to their outputs. AI is commonly recognised as a ‘general-purpose technology’: one which has potential applications across many sectors of the economy. It has general utility, because its skill – identifying patterns and making predictions – reflects the way humans produce all types of knowledge.
It is too soon to say whether, and when, AI will have a big impact on economy-wide productivity. There is a good case that it will, because it is explicitly designed to be labour-saving (as opposed to other tech innovations, such as social media and email, which can be a time sink). AI has already relieved humans of certain tasks – for example, allowing workers to read machine translations, which are often sufficiently accurate to avoid hiring translators – and is already helping to automate computer coding. Yet the various attempts to quantify the impact on jobs and GDP are highly speculative. In 2020, the World Economic Forum reckoned AI will take over 85 million jobs globally by 2025, but generate 97 million, which was spuriously precise, given the fact that the technology is still immature and its effects on labour markets are in their infancy.6 A survey of economists conducted by the Centre for Macroeconomics found that most thought AI would raise global growth from 4 per cent in recent decades to between 4 and 6 per cent, but this is just a guess.7
To achieve a sizeable impact on productivity growth there are many problems that AI needs to overcome. When asked questions to which they cannot find the answer, LLMs sometimes ‘hallucinate’, giving plausible sounding but fictitious responses. This has sparked a debate about whether the problem is inherent to the models themselves, or whether it can be fixed. Running AI is also expensive: it takes much more computer power for ChatGPT to answer a question than for Google to perform a traditional web search. And humans, especially those working in fields like law and medicine in which mistakes can be catastrophic, will have to supervise AI closely to ensure that any errors are found, and the model is behaving as expected. If humans no longer have to produce initial drafts of work, but are instead focused on identifying mistakes, there could still be big productivity gains. But this illustrates that the need for human labour in other tasks in the production process may still pose a bottleneck, limiting AI’s productivity potential.
If the past is any guide, new technology often takes a long time to raise productivity, because businesses need to find the best uses for it. The first personal computer, the Kenbak-1, was sold in 1971. But the manufacture and sale of business computing and software did not discernibly raise US productivity growth until 1995.8 In Europe, there was no equivalent jump in productivity growth, because there was weaker investment in computing and software, especially in key industries – finance, wholesale, retail and agriculture.9 There is intriguing evidence that more novel technologies with a potentially larger long-term impact, such as AI, may take longer to be put to practical use on an economy-wide basis.10
HOW EUROPE IS RESPONDING TO AI
The EU has only identified AI as a priority in recent years – and its openness to foreign AI providers has sometimes been equivocal. The European Commission’s 2015 Digital Single Market strategy did not mention AI, although its research and innovation programmes had already funded some AI projects. By the 2017 mid-term review of the strategy, however, the Commission had begun to identify AI as a potential driver of productivity growth, and set out an ambition for Europe to take a leading position in developing AI technologies, platforms, and applications. However, this was tempered by a fear of non-European firms dominating the sector: the Commission wanted the EU to “ensure Europe does not become dependent on non-European suppliers”.11
In 2018, the Commission and member-states agreed a common plan to accelerate the development of AI.12 The 2018 plan focused more on opportunities than supposed threats – the plan aimed to ensure companies could adopt AI, proposed measures to improve skills so workers could use the technology, and suggested guidelines to ensure AI development in Europe would be “responsible” and “ethical”. This was followed by a 2020 white paper which set out in more detail the EU’s plans to promote investment in AI and pointed towards the need for new regulation.13
In 2021, the Commission announced a concrete package of measures to promote AI and strengthen the EU’s capabilities, including an AI Act. The proposed Act is currently making its way through the EU’s institutions. The Act would set up a mostly risk-based framework for AI systems in use in the EU, and would apply equally to European or foreign-designed systems. The Commission’s proposal would prohibit the marketing of AI systems that pose the highest risks to consumers’ fundamental rights, such as systems which can subliminally manipulate people. For other high-risk AI systems, such as those used for biometric identification, the Act would make companies go through a conformity assessment and put in place various controls. Systems with less risk, such as back-end systems used to help optimise supply chains, would still need to meet some standards: for example, by making it clear to customers that they are dealing with an AI system and monitoring the system to make sure that any breaches of EU standards are logged and reported. The proposal mostly regulates firms which deploy AI (as set out in Chart 1 below), though it seems likely that MEPs, EU member-states and the Commission will agree to impose some obligations on developers of foundation models, regardless of how the models are actually used. Although there are problems with the Act (see below), in principle it could help drive take-up by giving users more confidence in the technology and enabling the EU to benefit from both home-grown and foreign AI models.
Some European businesses, however, want to make sure the EU builds the entire AI end-to-end supply chain rather than rely in part on foreign firms. For example, the German government recently commissioned a feasibility study for developing a European LLM. In the study’s preface, the president of the German AI Association, a network of German AI companies, warned that:
‘If we are not able to develop and deliver this basic technology on our own, German industry will have to shy away from foreign services, lose parts of the value chain and lose competitiveness.’14
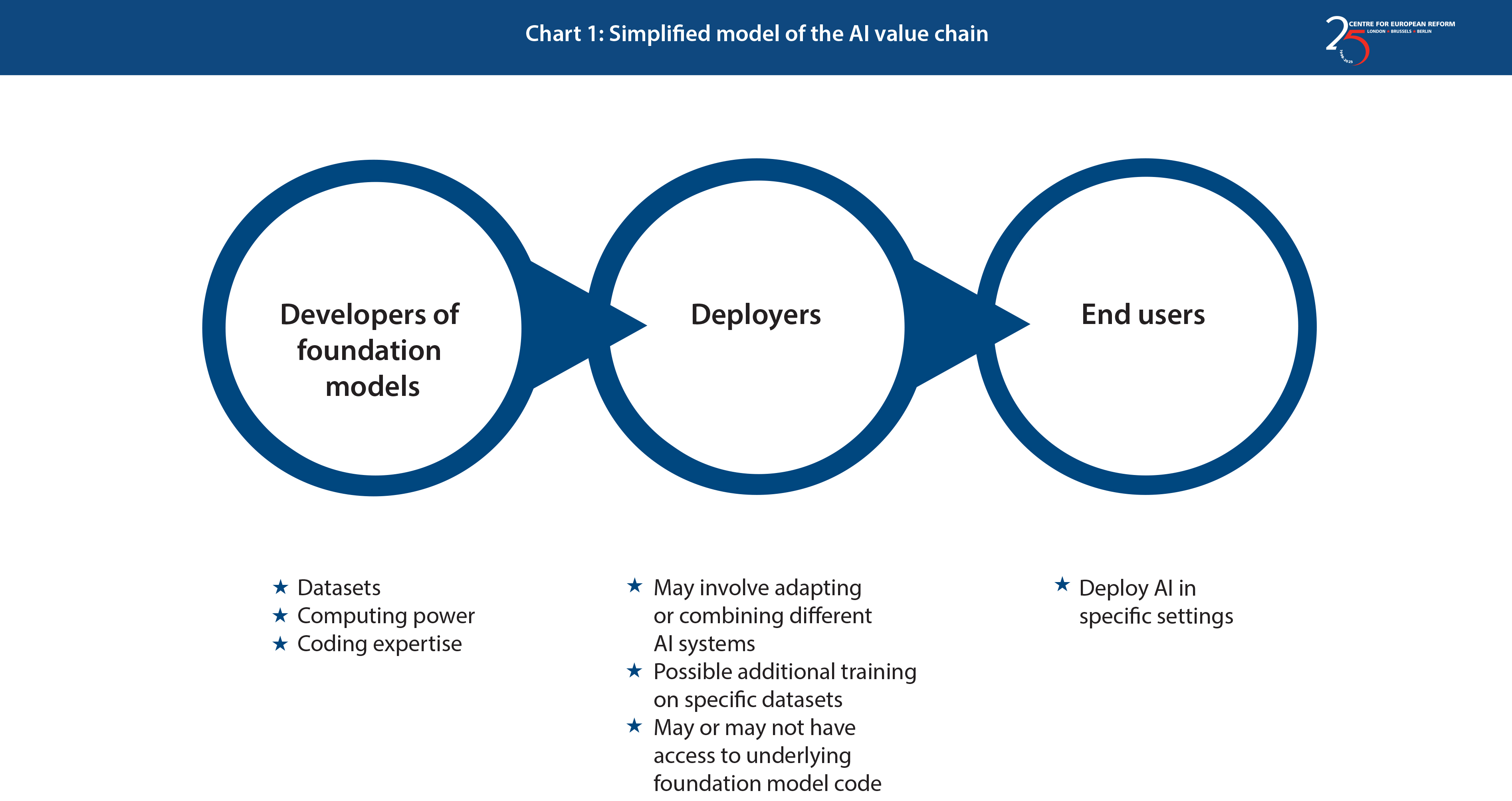
Currently, 73 per cent of large AI models are being developed in the US and 15 per cent in China.15 The Commission’s approach of setting standards for AI systems deployed in Europe, whatever the country of origin of the developer, is therefore sensible. The fact that most foundation models are American is not relevant from the perspective of European productivity. There is not necessarily much economic benefit for Europe in focusing on replicating foreign technologies rather than promoting the diffusion of technology. The UK, for example, has strong capabilities in basic research, but its productivity has plateaued for years because British businesses have failed to invest in using technology that already exists. Conversely, at the end of the 19th century, the US focused less on its own innovations and more on integrating European innovations into American industry – which proved a very successful strategy for US economic growth. Innovation is necessary for economies that are making full use of existing technologies. But European services firms have under-invested in technology for decades. This suggests the EU may be better off focusing on diffusing AI.
Reliance on foreign suppliers might pose a problem if they were excessively concentrated in any one country, especially if that country was a geopolitical rival to the EU. However, as Table 1 overleaf shows, although not every model has the same potential set of applications, there are already a large number of models on the market from a range of geographic regions. And many of the models are ‘open’, meaning their full source code and training data have been made available and the model is available for third parties to freely use, examine and build from.
Although most AI foundation models have not been developed in Europe, major non-English European languages are well represented in AI datasets. They represent 44 per cent of data from the 20 most used languages, with English, Spanish, French and German being the top four languages.16 Furthermore, numerous AI models have been built in or adapted to specific European languages. For example, Meta has released CamemBERT and FlauBERT, French versions of its BERT model. Meta also produced a multilingual model called NLLB-2000. BigScience has published a fully multilingual foundation model (BLOOM), which was produced in collaboration with French governmental agencies. AI Sweden has produced its own Swedish-language model, GPT-SW3, and LAION has produced several foundation models in Germany. German start-up Aleph Alpha has models which communicate in German, French, Spanish, Italian and English.
Given the potential for AI to raise Europe’s disappointing productivity growth, policy-makers should focus on ensuring that foundation models are tested and used by businesses rapidly. AI may disappoint, but it is only by testing its broader applications that we will discover their potential. To ensure the EU can do this, we propose four areas of focus:
- ensuring competition between AI foundation models, so that European businesses can be assured of fair prices and reasonable access to the technology;
- encouraging and supporting European businesses to use AI;
- ensuring the benefits of AI are fairly distributed, which in turn should help reduce resistance to it; and
- ensuring AI complies with European values, whether the suppliers are European or not.
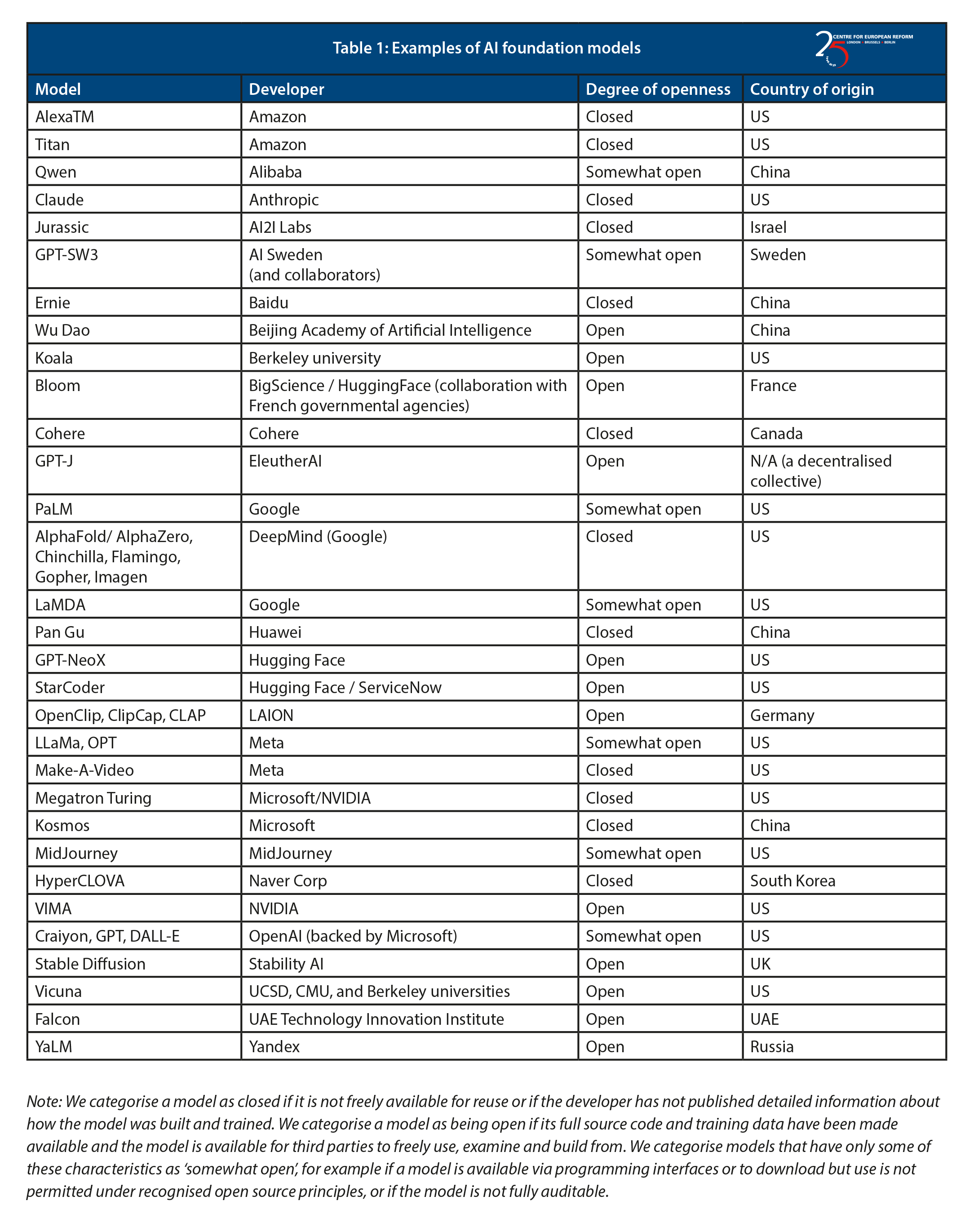
COMPETITION BETWEEN AI FOUNDATION MODELS
Competition between AI foundation models would give European businesses a broader range of choices at a fair price, and push AI companies to keep improving quality. The availability of more models provides more opportunities for businesses to tailor them to their own needs. It would also help ensure that the firms which produce AI models do not become so economically and politically powerful that they can easily disregard European values or the specific needs of their European customers.
In other tech markets, EU policy-makers have been concerned about industry concentration for years. Tech giants have introduced innovations that benefit European businesses and consumers. For example, Google and Facebook allow businesses to target advertising on consumers who are most likely to be interested in their products, which makes their advertising spending much more efficient. However, high market concentration means that too much of the benefit of some technologies is being retained by large tech firms, and too little is being passed onto customers. In competitive markets, innovative firms should enjoy a temporary period of high profits, as a reward for taking risk. Then, other companies should eventually catch up and exert competitive pressure, and the leading firm’s prices should drop as it focuses instead on diffusing its technology as widely as possible. This would normally increase productivity across the broader economy.
This dynamic has not always occurred. Instead, some large tech firms started with free and open services. But some of them have since achieved unassailable positions in certain markets, creating an economy dominated by a handful of superstar firms, who now have more freedom to increase prices, or reduce their openness, than they would have in a more competitive market.17 This suggests that, in some cases, the European economy – although it is better off than it would have been without large tech firms’ services – is not enjoying a fair share of the benefits. Furthermore, because these firms can sometimes become chokepoints for the digital economy, Europeans have worried that these firms may feel free – or be compelled by a foreign government – to disregard European values and interests. Could AI follow the same pattern?
Like other digital markets, AI has begun with a range of services, many of which are currently free to use, and which have – as Table 1 shows – a varying degree of openness. But it is not clear yet whether the market for AI foundation models will eventually coalesce around one or two players, or whether one or two foundation models might dominate each use for AI.
Today’s AI market is diverse and dynamic
Today there are many different foundation models on the market. Although the first LLMs only began to produce coherent text in 2019, in 2023 there is a multitude of providers. These include products made by, or financially backed by the familiar tech giants. But there are also many newcomers to the market. These include Anthropic, Cohere, Mistral, and the Elon Musk-backed xAI. Furthermore, as Table 1 shows, many AI models have a significant degree of openness. Not all ‘open’ AI models allow the same degree of transparency, reuse and ability for third parties to adapt and extend them.18 However, several firms nevertheless allow their models to be widely used by other developers. This can improve competition by making it easier for new firms to enter the market, since they can piggyback off the work of existing market players. Businesses may ultimately prefer open-source models over closed ones – for example, because they allow the way the underlying model works to be scrutinised, allow greater customisation for particular uses, and reduce the risk of being locked into one commercial proprietary model.
Many barriers to entry could prove surmountable
Some fear that, at first glance at least, barriers to entry in AI might appear high. As Chart 1 shows, building an AI foundation model requires significant computing power, access to training data, and skilled experts. Many models are therefore backed by deep-pocketed and patient investors. This poses some risks. For example, training, adapting and deploying AI requires a lot of computing power. In practice, this often requires players in the AI value chain to use one of the few large cloud computing companies – Amazon, Google and Microsoft. There are concerns about business users becoming ‘locked in’ to one cloud computing supplier,19 and European cloud computing firms cannot offer the same scale or resources as the US giants. Ninety per cent of the computing power behind all LLMs reportedly lies with seven large companies in the US and China,20 and 70 per cent of generative AI startups reportedly rely on Google’s cloud products.21
Yet barriers to entry and growth identified above may prove surmountable. Take computing power. Some of the EU’s recent initiatives aim to give European users more choice over bottleneck services like cloud computing, access to which will be essential for training AI models. The EU’s recent Data Act is also intended to help firms more freely switch between different cloud computing providers. And many national governments are supporting the construction of supercomputers which can help with the design and training of AI models, providing an alternative means of accessing computing resources. Europe has become a leader in supercomputing – with three of the five most powerful supercomputers in the world. And von der Leyen announced in her recent State of the Union speech a new initiative to open up our high-performance computers to help European AI start-ups to train their models.
Even apart from these initiatives, it is possible that the need for computing power will drop. Stanford researchers built an LLM based on Meta’s LLaMA for $600, and the costs involved in training models fall by roughly 60 per cent every year.22 So-called ‘small language models’ have been built by developers including Google (Albert), Meta (Bart), DeepMind (Retro), Baidu (Ernie), BigScience (T0) and Korean firm Kakao (KoGPT). Some apparently leaked internal Google memos show its employees fear that open-source models, in particular, can be built at far less cost than today’s large models and may nevertheless turn out to win large market shares:
“While we’ve been squabbling, a third faction has been quietly eating our lunch. I’m talking, of course, about Open Source. Plainly put, they are lapping us. Things we consider “major open problems” are solved and in people’s hands today... Open-source models are faster, more customisable, more private, and pound-for-pound more capable.”23
It is still unclear whether cheaper but more finely tuned models may ultimately offer superior results to more expensive models – for example, some users have observed a decline in quality in GPT-4, which is based on multiple small models rather than one large model like GPT-3. But there seem to be at least some use cases where smaller models could outperform big ones. This may allow smaller firms to remain competitive without access to mammoth computing power and financial resources.
Access to training data is not necessarily insurmountable either. Data is non-rivalrous – it can be used to train many different foundation models – and many foundation models are trained primarily on publicly available information, such as ‘Common Crawl’, a repository of publicly available internet content maintained by a non-profit organisation, and ‘The Pile’, another freely available dataset. Over 70 per cent of the data used to train Meta’s LLaMA, for example, was from internet scraping and Wikipedia.24 While fine-tuning models may require more specialised or high-quality data, this will not always be in the hands of large technology companies: an AI model trained to support lawyers, for example, might need to be trained on proprietary legal databases operated by specialist publication houses. And the EU institutions have in recent years tried to improve access to datasets held by the public sector, while encouraging – and in some cases mandating – private firms to share data more widely to create economic value.
Expertise is another area where large technology companies’ advantage may be overestimated. Many large technology firms have consistently struggled to maintain their best in-house AI experts, which means that competitors may be able to tempt them to join a promising new company. All but one of Google’s employees who co-authored a ground-breaking paper on AI have since left Google for OpenAI or later startups like Inflection AI and Adept AI.25
The biggest barrier to entry may instead be the global shortage of the graphic processing units (GPUs) essential for training AI. The market capitalisation of NVIDIA, a chip designer, has skyrocketed because of its unique position in supplying these chips. The firm is now more valuable than Meta. Furthermore, as the most cutting-edge GPUs are exclusively made in Taiwan, there are significant risks that access to high-end GPUs for AI would become even more constrained if there was an international conflict over Taiwan. But the competitive impacts of a conflict in Taiwan are very difficult to predict at this stage. One possibility is that only the richest companies would be able to afford chips, hindering smaller competitors. Another possibility is that the constraints would affect all firms, large and small, and simply hinder the overall development of AI.
‘Winner takes all' dynamics can be avoided
The business model of AI providers also suggests a possible race towards a ‘winner takes all’ outcome. Despite their high operational costs, many AI providers are currently providing services for free. This may be essential to stoke user demand and to help businesses and consumers see the benefits of a new technology. However, AI providers therefore need vast amounts of up-front capital and investors who are prepared to take risks without seeing an immediate path to profitability, raising the risk that only a handful of well-funded firms will make it through this phase of the race towards market maturity, and that European AI efforts, which are typically less well funded, will ultimately fail. Over 1.5 billion users visited the ChatGPT website in July 2023, but its owner, OpenAI, is said to be losing approximately $700,000 per day to operate the service, which has made it heavily dependent on Microsoft’s financial support.26 That may also explain why OpenAI abruptly stopped being a non-profit company committed to publishing its AI software as open source – and suggests that not every AI firm operating today may have a sustainable business.
A ‘winner takes all’ outcome would also be more likely if AI foundation models enjoyed big economies of scale, where output rises faster than capital and labour costs as firms grow, making it hard for competitors to catch up. Or there might be significant network effects, whereby a service becomes more attractive the more users adopt it. These have been features of many other digital services, and have contributed to a dynamic where the most popular service, or the one with the most early investment, becomes difficult to dislodge. For example, some analysts believe Google’s large market share in online search results from it having more users than alternatives, which in turn gives it more user feedback than its competitors, which it can use to further improve its results.27 AI services may have a similar feedback loop, which may explain why Meta adopted an open-source approach: maximising users may then give its model more data and feedback to work with, which the model can then use to hone its capabilities. There is evidence from other tech markets of firms adopting an ‘open’ approach to help gain scale and then adopting a ‘closed’ approach once they become dominant. These suggest ‘winner takes all’ outcomes that are likely to benefit just a few American firms.
Over time, if ‘winner takes all’ dynamics exist, the AI sector may consolidate, and start-ups may be founded merely with a view to being acquired. That means competition regulators will need to be careful to scrutinise mergers and acquisitions (M&A) activity, or regulation may be required to address the underlying market dynamics that promote consolidation. At the moment, however, the sector seems sufficiently diverse and dynamic that acquisitions should not be presumed to be anti-competitive. And regulators seem alive to the risks of excessive consolidation. EU and UK antitrust authorities have recently shown a willingness to block deals in innovative and fast-moving sectors, based on their judgement of future market developments.28 These prohibitions make M&A activity in high-tech sectors much more risky today than it was in the past. That suggests investors in AI start-ups are not necessarily counting on the firm being acquired by ‘big tech’ – but may instead see AI firms as having valuable sustainable potential as competitors to the giants.
Leveraging
Finally, large tech firms may try to use their existing dominant products to provide an unassailable advantage to their own AI models – for example, by only allowing their own AI services to run on their operating system. These concerns can arise even if the models are open source: the European Commission charged Google for acting anti-competitively in relation to its open-source Android operating system. Firms may also use their influence or control over other parts of the supply chain to gain advantages. For example, AI developers rely on just a few development frameworks – sets of pre-built software components – to help build their models. The two most important, PyTorch and TensorFlow, have connections with Meta and Google respectively, which means either company could seek to influence how the framework develops in order to promote their own services. These types of practices, which leverage different markets or parts of the supply chain, can take many forms, and it is not always easy to identify whether a particular practice promotes innovation or has a long-term negative impact on competition. This creates the risk that competition authorities will only intervene in such practices once it is too late.
Although there is potential for anti-competitive conduct from ‘leveraging practices’ – where tech giants lock in users to their pre-existing products – antitrust authorities have become significantly more vigilant about that in recent years, and have a number of new tools they can use to help keep AI markets open and competitive. The EU’s Digital Markets Act, for example, will address many of the ways firms have built ecosystems, for example by preventing them from stopping users from freely switching between different apps and services. Microsoft, one of the largest AI firms, has also shown its willingness to adjust its business model to reflect some of the concerns about its leveraging practices. For example, in August it agreed to stop bundling Office 365 and Teams together. Given the pace of change in AI, regulators will also need to have the digital and data skills necessary to monitor and understand market developments and prevent anti-competitive practice.
Prospects for competition: Too early to judge
Ultimately, it is unclear how much competition between foundation models will be sustainable in the long run. The market is nascent, with a lot of experimentation going on, and it is too early to properly quantify the impact of barriers to entry, economies of scale and network effects. For now, EU competition authorities should keep a close eye on market developments, and ensure they have the tools and expertise to act quickly if evidence emerges that competition is falling and European businesses do not have adequate access to AI. The UK Competition and Markets Authority’s study of AI foundation models, which will be published mid-September 2023, illustrates how regulators can build a working knowledge of the technology and its potential impact.
Ensuring AI remains widely available will have important impacts within European markets – especially if European firms focus on adapting and deploying AI, rather than building their own foundation models. If the cost of adapting and deploying models is low, then European markets are likely to become more dynamic, with new entrants in AI deployment, and users in wider manufacturing and services sectors, able to use AI to deliver rapid productivity gains and to quickly pivot into new markets. That is likely to help European businesses become more innovative and European consumers to enjoy cheaper, higher quality and more cutting-edge products and services.
ENCOURAGING TAKE-UP
Competition will ensure that AI foundation models are available for European businesses to use. However, that will not ensure that European businesses adopt and exploit the technology.
As noted above, many ‘general purpose’ technologies take a long time to be put to use. In the past, that has been because infrastructure upgrades were needed – new factories needed to be redesigned to take full advantage of new machinery, and more recent technologies have required the rollout of new electronic communications networks and cloud computing services. AI requires high-end chips, cloud computing services, data centres and sufficient energy to run them – and policy-makers should help ensure these do not pose barriers to take-up. But in general, firms that want to use AI do not typically need to invest in massive new infrastructure, in the form of new cabling for example, and this may hasten its spread. Although some European countries have underinvested in infrastructure and digitalisation, many EU member-states have widespread fibre-based networks, which give businesses virtually limitless connectivity, and data centres with massive computing power (although many more will have to be built if AI takes off). However, given EU firms’ lethargy in integrating new technologies, the EU and its member-states should consider reforms that would help nudge them to adopt the new AI technology more quickly.
Support research and deployment
First, policies can directly support AI adoption in the EU. These initiatives could include more public research and development grants for AI, and more tax incentives for businesses to invest in AI deployment. AI research centres could also help improve collaboration across businesses, and with academia and public bodies, to help promote knowledge exchange and technology transfer. The EU can provide guidance on such tax incentives to its member-states, and support research centres through its grants, to ensure a level playing field across the single market. Yet currently the EU’s Digital Decade policy programme has surprisingly little to say about AI – focusing instead on projects like semiconductor manufacturing, high-performance computing, and quantum technologies.
Invest in skills
Second, a core constraint remains the lack of digital skills in the EU: over 70 per cent of EU businesses cite a lack of digitally skilled staff as an obstacle to making further ICT investments. Small and medium enterprises, in particular, have struggled for years to attract the ICT professionals they need. Addressing this problem would require a combination of encouraging the immigration of workers with digital skills, and investment in education and training to help develop a skilled AI workforce. Here, the Digital Decade programme, and numerous member-state policies, recognise the scale of the challenge. However, as Chart 2 shows, in recent years the EU has made very little progress in increasing ICT-related employment, which remains far below the EU’s target for 2030 (10 per cent of total employment). And the proportion of ICT graduates has barely changed, which suggests this problem will persist.
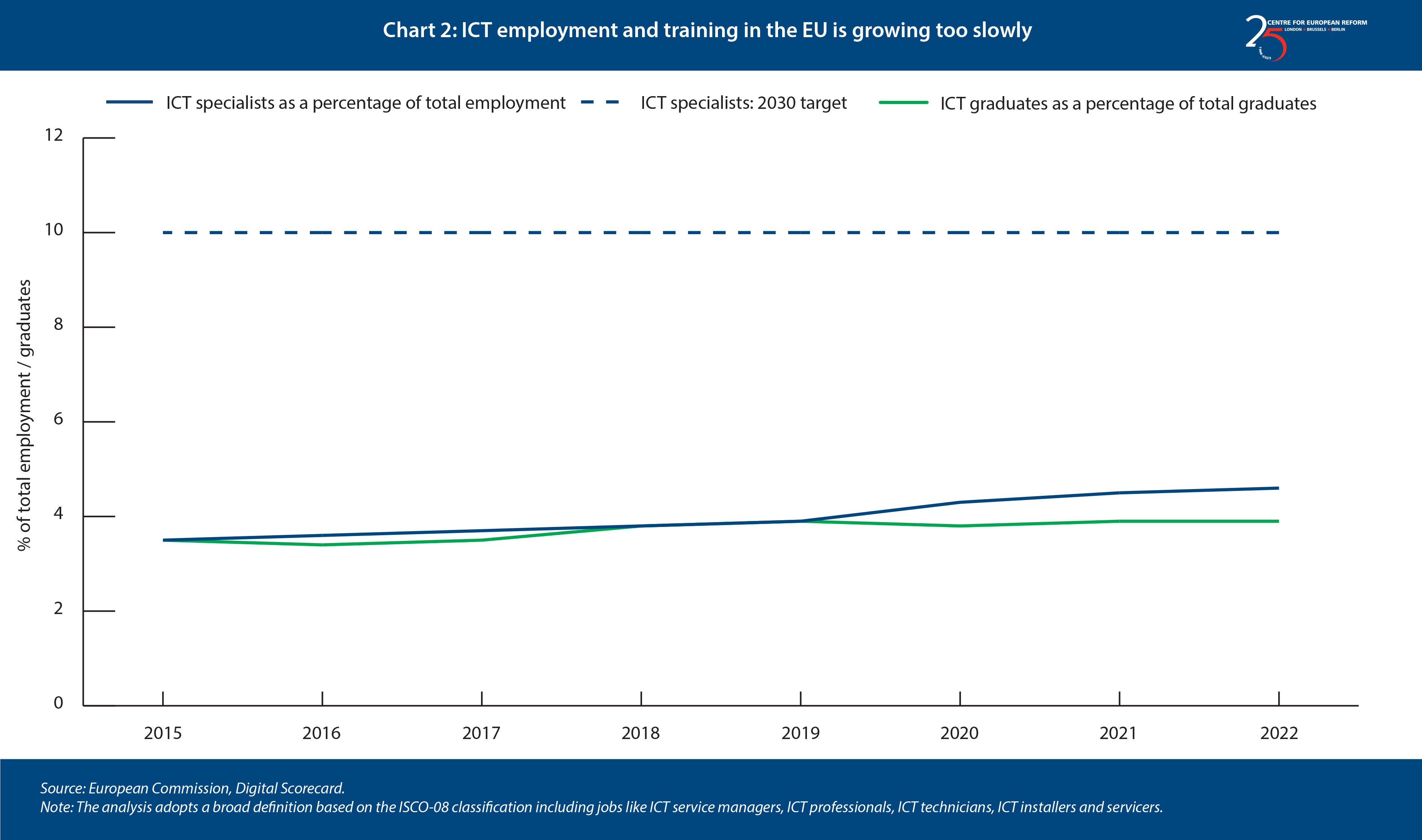
Ensure regulation and regulators are AI-ready
The other major barrier to business take-up of AI is regulatory: businesses may fear AI might expose them to risks, such as liability if an AI model has breached a person’s intellectual property rights, or if the AI model’s output is inaccurate, defamatory or discriminatory.
The law already addresses many of these issues: for example, EU or member-state laws cover IP rights, discrimination, defamation, and harmful content. And the EU has recently passed a number of laws, like the Digital Services Act, which address some of the most important risks – for example, that online platforms’ AI-fuelled algorithms might inadvertently propagate disinformation or interfere in elections.
However, it is not always clear how these laws apply to AI. To list just some of these questions: how can AI models use data which is publicly available online but protected by data protection or intellectual property laws?29 If models have not complied with the EU’s data protection laws in using training data, must the models be fully retrained on compliant data, or does GDPR only regulate the consequent outputs of AI models? Who, if anyone, owns the intellectual rights in the output of AI models? What liability should providers of foundation models have for decisions made by their customers? What degree of oversight, accountability, and ability to explain outcomes should AI systems have?
Businesses are unlikely to take up AI while these questions are not fully resolved. EU and member-states’ legal frameworks therefore need to become ‘AI-ready’. In many cases, this can be resolved by regulators and public authorities issuing new guidance and policies on how existing laws apply to AI, rather than overhauling the law or creating new laws. In addition to clarifying how the law applies, public authorities and regulators will also need to be ready to apply the law to contexts that involve AI. To do so, they will need to increase their digital literacy, employing more individuals with skills in data science and coding, and they may need increased budgets to cope with the complexity of cases involving AI.
HOW TO REGULATE AI
However, even if existing laws address many of the problems AI could cause, there will remain many possibilities for AI to cause damage without breaking the law, and its diverse capabilities mean that it could quickly introduce new risks. This could make businesses reluctant to take up AI.
To address this, potential users of AI will want reassurance that AI providers will act responsibly and that new risks will be identified and addressed quickly. Many large AI providers’ have made public commitments to act responsibly.30 Furthermore, there are a range of international efforts to establish ‘rules of the road’ for AI, including through international forums like the G7 and the OECD. At this stage, most of the measures are voluntary and not directly enforceable. They go some way to instilling trust in the technology, but because these measures primarily rely on businesses trusting that AI providers will comply with their commitments, voluntary commitments disproportionately benefit well-established firms with good reputations. That could hamper the opportunities of the many smaller start-ups in the AI sector.
There is therefore an important role for regulation. Some smaller AI firms may find it tough to comply with mandatory rules. However, compulsory rules will in the long run put smaller and larger firms on a more equal footing in terms of public trust. Such regulation does, however, need to be proportionate in order not to make it more difficult than necessary for new firms to launch AI services. Regulation should, for example, require less accountability for uses of AI that have relatively low risk – which will include many productivity-enhancing uses of AI. Regulation should also build on and be compatible with globally agreed rules and principles, to avoid adding unnecessary burdens to businesses who want to operate across borders.
The European Commission’s recent proposal for an AI Act provides a reasonable basis for addressing higher-risk uses of AI. The AI Act adds to existing laws, by putting in place a new framework that is primarily focused on ensuring AI providers are accountable and manage risks appropriately, rather than fitting AI into existing regulatory regimes.
However, the AI Act also poses a number of problems.
First, while adding a new layer of accountability is useful, the Act does not perform the job of clarifying how existing laws apply to AI, for example how to comply with the GDPR or who is liable if AI produces unlawful output. These are likely to fall within both EU and member-state competences. Reviewing and providing guidance on existing laws and regulations – which would ideally be done by way of a comprehensive review, encompassing both EU-level and member-state rules – will be necessary to give AI developers more certainty about how the law applies to AI. The AI Act would also benefit from allowing more useful ‘regulatory sandboxes’. These allow firms to test, develop and deploy AI in a controlled environment, where the model can be tested on users before a full commercial launch, thereby avoiding the risk of breaking existing regulations. Such sandboxes have worked well at encouraging innovation in some other sectors, such as financial services. However, the currently proposed form of sandbox in the AI Act is very limited and should be expanded: for example, the sandbox rules only help firms ensure compliance with two specific laws (the AI Act itself and the EU’s data protection laws) without clarifying how AI testing can be compatible with a wide range of other EU laws.31
Second, the AI Act does not offer a very specific definition of AI, referring only to general concepts like software that can “generate outputs” using techniques like statistical approaches. This definition has the potential to be unhelpfully broad. While the European Parliament and member-states have proposed more narrow definitions, for example by requiring that the system operate with “varying levels of autonomy”, there is still potential for the AI Act to regulate technologies and systems (like the algorithms which drive Google search today) that are already well established and are not typically considered to pose new risks. Currently, EU businesses have options to deploy AI systems without any regulatory compliance burden – but under law-makers’ proposals, almost all uses of AI would involve some additional compliance costs, even if the system has been in place for years. If it inadvertently regulates existing technologies with low risk, the AI Act may make their adoption more difficult for no good reason, or even encourage firms to ditch technologies they have already adopted.
Third, the Commission’s AI Act proposal does not clearly delineate responsibility across the AI value chain. The Commission’s original proposal imposed different obligations on different types of AI systems based on the risks they pose, and would have placed most regulatory responsibility on the deployer of an AI system rather than its developer. This would put European businesses who want to use AI in a difficult position. For one thing, foundation models are not limited to particular applications, and are user-friendly enough to be operated by anyone, so their level of risk is indeterminate. For another, European businesses that primarily want to adapt or deploy foreign firms’ foundation models may not be in a good position to mitigate many of the risks: after all, they will not necessarily have access to the foundation model’s source code or training data. For example, taking the foundation models set out in Table 1 (page 6), there are a range of different business models, ranging from:
- closed, ‘proprietary’ foundation models which offer limited transparency about the underlying algorithms or training data, and where the foundation model’s developers can restrict its use and output;
- models that are accessible on the cloud, and offer more transparency, while still retaining control over consumers’ use and the types of output from the model;
- downloadable and open-source models, whose source code and training data can be scrutinised by anyone, which can be freely retrained with additional data, and whose end uses cannot be constrained through technical means.
It seems clear that law-makers are likely to agree to impose some regulatory obligations on most or all providers of foundation models. However, the complexity and variety of business models mean that a ‘one-size-fits-all’ approach will not work. For example, imposing obligations on the developers of open-source foundation models will not address the risk that businesses may tweak or use that model in ways which would breach the AI Act. But it would be inappropriate to impose onerous obligations on deployers of foundation models in circumstances where they do not know what is in the underlying foundation model’s code or initial dataset.
Law-makers are currently negotiating how to adapt the AI Act proposal in light of new services like ChatGPT. In doing so, they will need to keep in mind the productivity benefits of keeping the technology widely available in the EU, and the benefits of sticking with a risk-based approach – after all, many of the most productivity-enhancing uses of the technology will occur when AI is deployed in activities like document drafting, record-keeping, and optimising business processes. As over 150 European CEOs recently said,32 imposing a high ‘baseline’ level of regulation on providers of foundation models – even if the model is only used for a low-risk purpose – risks hampering innovation and increasing the productivity gap between the EU and the US.
If these problems can be fixed, the principle of an EU-wide AI Act is sound. The Act would give European standards bodies the power to interpret its requirements, which should ensure firms have pragmatic and proportionate ways to prove their compliance. The act could also raise trust in the technology and provide a clear and consistent set of rules across the EU to avoid regulatory fragmentation – making it cheaper and easier for European businesses to roll out AI at scale.
A final problem is that many foreign governments do not comply with European data protection standards – for example, some foreign national security agencies can obtain access to data about EU nationals held by their country’s cloud computing companies. In response, the EU is making it increasingly difficult for European firms to use some foreign digital services. The EU’s cloud cybersecurity certification scheme for cloud services is likely to prevent foreign cloud computing providers (and AI services that rely on them) from achieving the highest security certification, which means European firms will be less comfortable using them. This could pose a serious barrier to European firms using foreign AI services, since such services typically require access to large cloud computing platforms, and very few of these platforms are European.
These requirements are ill-conceived because they take a black-and-white approach to one particular risk – namely, foreign government access to data – rather than adopting a more holistic assessment of the overall cybersecurity and privacy risks of using particular foreign suppliers. The EU seems unlikely to step back from these requirements, which will probably raise the costs of using foreign AI models in Europe and constrain their widespread deployment. Given many of the most important AI and cloud computing systems are American, long-term EU-US dialogue to help bridge data protection requirements offers the best way forward. The recent EU-US Data Privacy Framework (the third attempt by the US to protect EU nationals’ data in a manner which will allow seamless transatlantic data transfers) illustrates that such dialogue can bear fruit.
CONCLUSION
Although consumers are already enjoying many uses of AI, its development is just beginning. Given the US, rather than the EU, is at the technological frontier, the EU’s AI strategy – focussing on ensuring European businesses exploit the technology – is a sound way to improve Europe’s productivity and economic growth. This will require competition authorities to scrutinise the market carefully to avoid one or two providers of AI foundation models becoming dominant (though reasons to fear this outcome are limited at the moment). It will also require policy-makers to undertake a broad review of how AI can comply with existing regulatory regimes, to give European businesses more certainty and nudge them to move ahead with adoption. With some adjustments, the proposal for an EU AI Act could be a useful step towards a consistent EU-wide approach, but a much more expansive review of existing laws is needed.
Even if competition between AI foundation models is thriving and government policies support take-up, an important source of resistance to adopting AI in Europe will remain: fears about its distributional impacts, in particular the way in which AI may displace certain workers or entire types of work.
In the past, technological disruptions like automation in the manufacturing sector – even though they have had a positive transformational impact on the economy in the long run, and have helped the EU sustain its international competitiveness – have had concentrated negative effects on particular types of workers. But, these changes were often slow and initially uncertain, as it took time for businesses to work out how to fully exploit new inventions. The same will be true of AI. However, given that the EU is lagging in the global race for digital technology, and has lower productivity in some sectors than other advanced economies, the EU cannot afford to be a laggard. The EU’s biggest economic risk stems not from the deployment of AI – but that it fails to adopt it and drifts further down the economic league table.
No comments:
Post a Comment