Owen Hughes
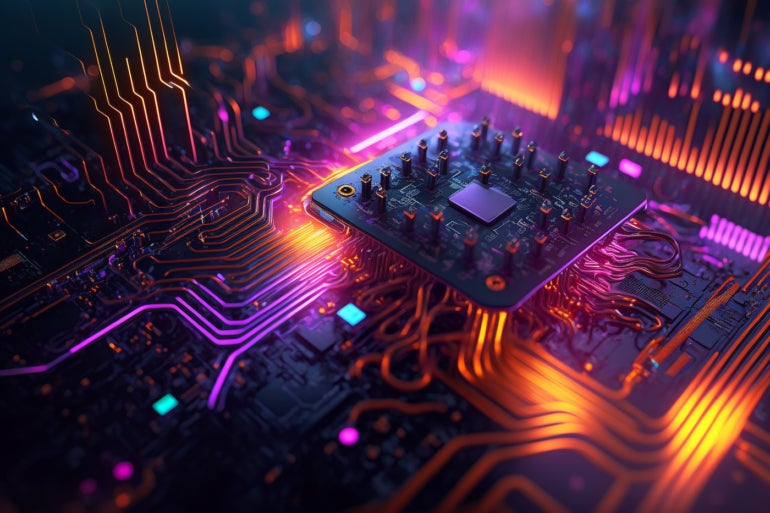
What is generative AI?
In simple terms, generative AI is a subfield of artificial intelligence in which computer algorithms are used to generate outputs that resemble human-created content, be it text, images, graphics, music, computer code or otherwise.
In generative AI, algorithms are designed to learn from training data that includes examples of the desired output. By analyzing the patterns and structures within the training data, generative AI models can produce new content that shares characteristics with the original input data. In doing so, generative AI has the capacity to generate content that appears authentic and human-like.
How does generative AI work?
Generative AI is based on machine learning processes inspired by the inner workings of the human brain, known as neural networks. Training the model involves feeding algorithms large amounts of data, which serves as the foundation for the AI model to learn from. This can consist of text, code, graphics or any other type of content relevant to the task at hand.
Once the training data has been collected, the AI model analyzes the patterns and relationships within the data to understand the underlying rules governing the content. The AI model continuously fine-tunes its parameters as it learns, improving its ability to simulate human-generated content. The more content the AI model generates, the more sophisticated and convincing its outputs become.
Examples of generative AI
Generative AI has made significant advancements in recent years, with a number of tools capturing the public attention and creating a stir amongst content creators in particular. Big tech companies have also jumped on the bandwagon, with Google, Microsoft, Amazon and others all lining up their own generative AI tools.
Depending on the application, generative AI tools may rely on an input prompt that guides it towards producing a desired outcome — think ChatGPT and DALL-E 2.
Some of the most notable examples of generative AI tools include:ChatGPT: Developed by OpenAI, ChatGPT is an AI language model that can generate human-like text based on given prompts.DALL-E 2: Another generative AI model from OpenAI, DALL-E is designed to create images and artwork based on text-based prompts.Google Bard: Google’s generative AI chatbot was rolled out as a competitive response to ChatGPT. It is trained on the PaLM large language model.Midjourney: Developed by San Francisco-based research lab Midjourney Inc., Midjourney interprets text prompts and context to produce visual content, similar to DALL-E 2.GitHub Copilot: An AI-powered coding tool created by GitHub and OpenAI, GitHub Copilot suggests code completions for users of development environments like Visual Studio and JetBrains.xAI: After funding OpenAI, Elon Musk departed from it and announced this AI research project.
Types of generative AI models
There are several types of generative AI models, each designed to address specific challenges and applications. These generative AI models can be broadly categorized into the following types.
Transformer-based models
These models, such as OpenAI’s ChatGPT and GPT-3.5, are neural networks designed for natural language processing. They’re trained on large amounts of data to learn the relationships between sequential data — like words and sentences — making them useful for text-generation tasks.
Generative adversarial networks
GANs are made up of two neural networks, a generator and a discriminator, that work in a competitive or adversarial capacity. The generator creates data, while the discriminator evaluates the quality and authenticity of said data. Over time, both networks get better at their roles, leading to more realistic outputs.
Variational autoencoders
VAEs use an encoder and a decoder to generate content. The encoder takes the input data, such as images or text, and simplifies it into a more compact form. The decoder takes this encoded data and restructures it into something new that resembles the original input.
Multimodal models
Multimodal models can process multiple types of input data, including text, audio and images; they combine different modalities to create more sophisticated outputs. Examples include DALL-E 2 and OpenAI’s GPT-4, which is also capable of accepting image and text inputs.
Benefits of generative AI
The most compelling advantage generative AI proposes is efficiency, in that it can enable businesses to automate specific tasks and focus their time, energy and resources on more important strategic objectives. This often results in lower labor costs and an increase in operational efficiency.
Generative AI can offer additional advantages to businesses and entrepreneurs, including:Easily customizing or personalizing marketing content.
Generating new ideas, designs or content.
Writing, checking and optimizing computer code.
Drafting templates for essays or articles.
Enhancing customer support with chatbots and virtual assistants.
Facilitating data augmentation for machine learning models.
Analyzing data to improve decision-making.
Streamlining research and development processes.
Use cases of generative AI
Despite generative AI still being in its relative infancy, the technology has already found a firm foothold in various applications and industries.
In content creation, for instance, generative AI can produce text, images and even music, assisting marketers, journalists and artists with their creative processes. In customer support, AI-driven chatbots and virtual assistants can provide more personalized assistance and reduce response times while reducing the burden on customer service agents.
Other uses of generative AI include:Healthcare: Generative AI is used in medicine to accelerate the discovery of novel drugs, saving time and money in research.
Marketing: Advertisers use generative AI to craft personalized campaigns and adapt content to consumers’ preferences.
Education: Some educators use generative AI models to develop customized learning materials and assessments that cater to students’ individual learning styles.
Finance: Financial analysts use generative AI to examine market patterns and predict stock market trends.
Environment: Climate scientists employ generative AI models to predict weather patterns and simulate the effects of climate change.
Dangers and limitations of generative AI
It’s important to note that generative AI presents numerous issues requiring attention. One major concern is its potential for spreading misinformation or malicious or sensitive content, which could cause profound damage to people and businesses — and potentially pose a threat to national security.
These risks have not escaped policymakers. In April 2023, the European Union proposed new copyright rules for generative AI that would require companies to disclose any copyrighted material used to develop these tools. Hopes are that such rules will encourage transparency and ethics in AI development, while minimizing any misuse or infringement of intellectual property. This should also offer some protection to content creators whose work may be unwittingly mimicked or plagiarized by generative AI tools.
The automation of tasks by generative AI could also affect the workforce and contribute to job displacement, requiring impacted employees to reskill or upskill. Additionally, generative AI models can unintentionally learn and amplify biases present in training data, leading to problematic outputs that perpetuate stereotypes and harmful ideologies.
ChatGPT, Bing AI and Google Bard have all drawn controversy for producing incorrect or harmful outputs since their launch, and these concerns must be addressed as generative AI evolves, particularly given the difficulty of scrutinizing the sources used to train AI models.
Generative AI vs. general AI
Generative AI and general AI represent different aspects of artificial intelligence. Generative AI focuses on creating new content or ideas based on existing data. It has specific applications and is a subset of AI that excels at solving particular tasks.
General AI, also known as artificial general intelligence, broadly refers to the concept of AI systems that possess human-like intelligence. General AI is still the stuff of science fiction; it represents an imagined future stage of AI development in which computers are able to think, reason and act autonomously.
Is generative AI the future?
It depends on who you ask, but many experts believe that generative AI has a significant role to play in the future of various industries. The capabilities of generative AI have already proven valuable in areas like content creation, software development and healthcare, and as the technology continues to evolve, so too will its applications and use cases.
That said, the future of generative AI is inextricably tied to addressing the potential risks it presents. Ensuring AI is used ethically by minimizing biases, enhancing transparency and accountability and upholding data governance will be critical as the technology progresses. At the same time, striking a balance between automation and human involvement will be crucial for maximizing the benefits of generative AI while mitigating any potential negative consequences on the workforce.
No comments:
Post a Comment