DEVAKI RAJ and JAKE HARRINGTON
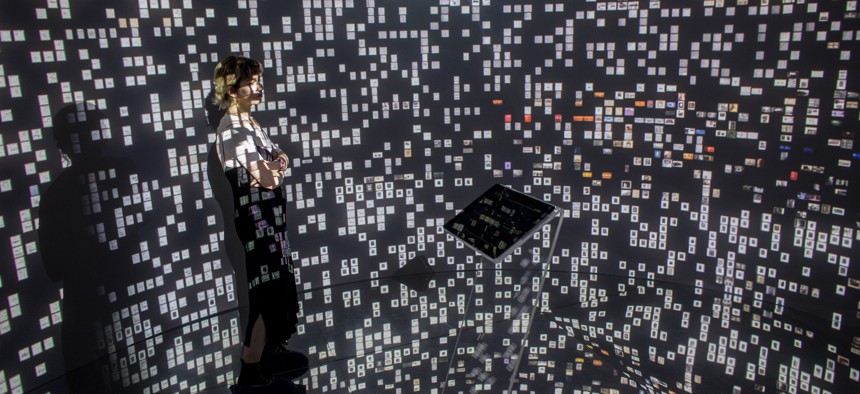
The Defense Department has issued strategy and guidance to transform itself into a “data-driven organization” and assigned smart, capable people to achieve this vision through multiple centers of excellence. But DoD must also cultivate a broader, enterprise-level understanding of modern software development and the fundamental realities of operational artificial intelligence platforms. In particular, DoD and other national security components must recognize the indispensable role of the end user in designing, developing, implementing, and iterating AI capabilities.
Based on our analysis of the government’s approach to software, and our experience building these capabilities in the private sector and with the Pentagon, we argue there are two areas where DoD should dedicate near-term attention. First, the actual end users of AI platforms must be closely involved in all aspects of the development process, from identifying and providing data to providing feedback on model performance. Second, DoD should implement an enterprise-level development pipeline for AI: an end-to-end solution, from data management to final model deployment, that serves as the primary mechanism for facilitating close, ongoing interaction between users and developers.
In our experience, government agencies typically outsource AI development to vendors, and make few efforts to involve the people who will ultimately use the tools. But users are best positioned to answer questions about what data is most relevant to the mission, and to assess the relevancy, quality, and quantity of various data sources that could contribute to the model. Often, they have painstakingly and tediously sought to normalize and analyze this data. They also know the quality of the data, and its overall usability. For data to be high quality, it not only needs to be relevant, but labeled well. Figuring out how to label data in a way that would provide the most value to users who will actually be using the AI models requires their close and ongoing involvement. End-users know what they are looking for in data and are best suited to define the ontology and to curate positive and negative training data.
While agencies often have lots of data, it is often not diverse. Having a thousand examples of the same thing is not all that helpful. Here too, users can be helpful when it comes to coordinating collection requirements to get plentiful, diverse data.
User involvement is also paramount when it comes to training, testing, and deploying production models, as they can offer real-time and feedback on model performance and utility. This constant feedback can be incorporated into retraining models to improve performance and utility.
The best way to facilitate user engagement is through an end-to-end AI infrastructure pipeline that does not require users to have knowledge of coding or machine learning. These types of low-or-no code tools are increasingly being embraced by the national security community, from CIA’s “Citizen IT” initiative to DoD’s data science cookbook. Given the importance of more fully integrating mission users into tool development, compounded by the reality that high end data science expertise will remain a scarce government resource, efforts to leverage personnel with “hobby grade” or even no data analytic skills will be increasingly important.
An end-to-end infrastructure pipeline would serve as a one-stop shop for data management and labeling as well as model training, testing, evaluation, and deployment. This pipeline would need to be largely code-free to integrate the essential expertise of non-technical users. It should also be available via cloud, on-premises, or at the edge, and across multiple security classification enclaves, in order to account for the various work environments across the defense enterprise.
A data management tool is an essential component of this pipeline, as it ensures data accessibility. Data needs to be relevant, high quality, and plentiful, but it also needs to be easily discovered and accessible for mission users to do anything with it. Unfortunately, national security data is often siloed between, and sometimes even within, organizations due to security, policy, and technological barriers. A data management tool, as part of a unified infrastructure pipeline, would help break these barriers.
A data annotation tool that automates the labeling of data after initial input from users would also speed up the essential process of labeling data. Labeled data would be managed in a data repository for future use and for use across the entire enterprise.
Intuitive model training, test and evaluation, deployment, and a user feedback mechanism will enable users to not only be involved in the essential, iterative aspects of AI development, but will make them the actual owners of it.
We have little doubt that U.S. leaders recognize the importance of artificial intelligence in securing our future strategic advantage. Essential within their efforts will be enlisting and empowering more and more of the workforce—the people who work hands-on with an organization’s data on a day-to-day basis—to drive AI and machine learning outcomes. As we have often heard from stakeholders across the government, technology, and academic spheres, technology is not the hard part in achieving DoD’s data vision. It will be the efforts to empower the right people and modernize the right processes that will prove instrumental in bringing about the Pentagon’s digital transformation.
No comments:
Post a Comment