Chris Baber, Ian Apperly, Emily McCormick
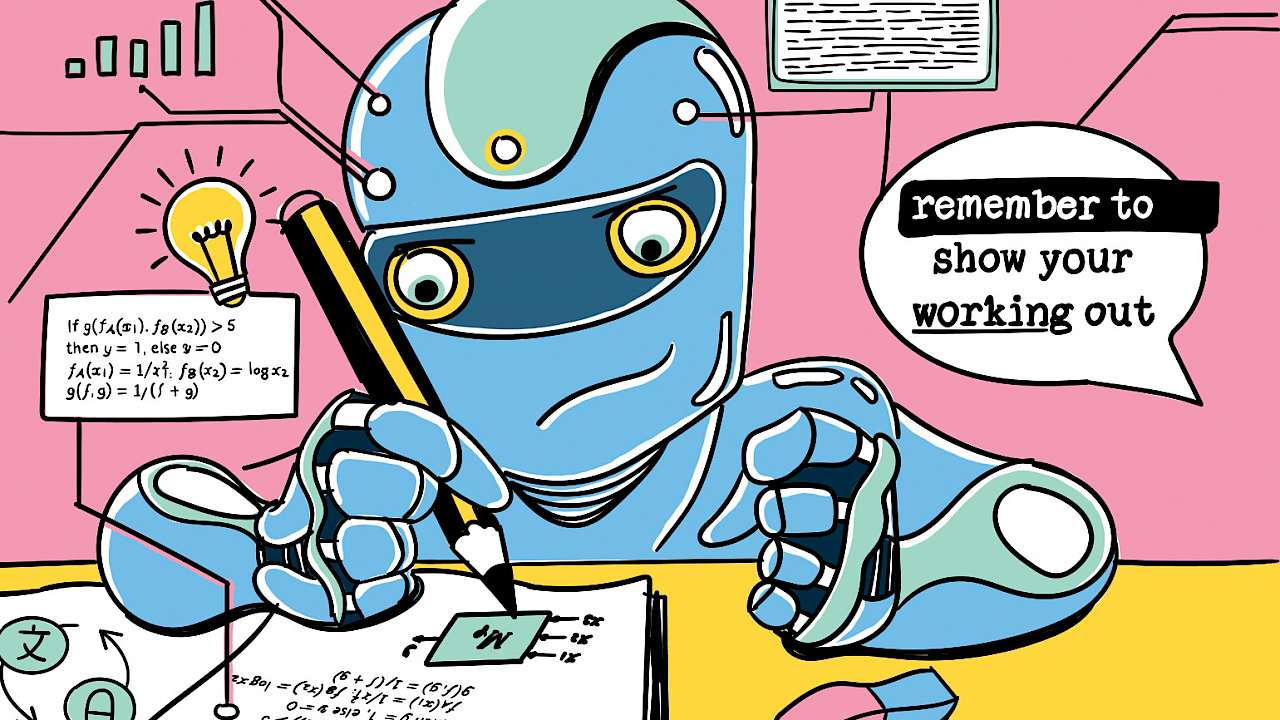
Executive summary
For artificial intelligence/machine learning (AI/ML) to augment human intelligence (in terms of extending a human’s cognitive capabilities through the provision of sophisticated analysis on massive data sets), there needs to be sufficient common ground in the way humans and AI/ML communicate.
In this report, we assume that interactions between humans and AI/ML occur in a system in which cooperation between humans and AI/ML is one interaction among many, e.g. humans cooperate with other humans; humans programme the AI/ML; humans could be involved in selecting and preparing the data that the algorithms use; the AI/ML could interact with other algorithms etc.
Not only is it important that humans and AI/ML establish common ground, but also that humans who communicate with each other using AI/ML share this common ground.
Explanation is the process by which common ground between interactions is established and maintained
From this perspective, the term ‘explanation’ is the process by which common ground between interactions is established and maintained.
We have developed a framework to highlight this concept, and this is instantiated to show how different types of explanation can occur, each of which requires different means of support.
Primarily, an explanation involves an agreement on the features (in data sets or a situation) which the ‘explainer’ and ‘explainee’ pay attention to and why these features are relevant.
We propose three levels of relevance:
‘Cluster’ – In which a group of features typically occur together
‘Belief’ – which defines a reason as to why such a cluster will occur
‘Policy’ – which justifies the belief and relates this to action.
Agreement (on features and relevance) depends on the knowledge and experience of the explainer and ‘explainee’, and much of the process of the explanation involves ensuring alignment between parties in terms of knowledge and experience.
We relate the concept of explanation developed here to concepts such as intelligibility and transparency in the AI / ML literature and provide guidelines that can inform decisions on the development, deployment, and use of AI/ML in operational settings.
From the framework of explanation developed in this report, we propose the following guidelines:
Explanations should include relevant causes. Explanations should relate to beliefs in the relationship between features of a situation and the causes that can directly affect the event being explained (probability) or can explain most of the event (explanatory power); are plausible (construct validity); and if the cause was instigated by a person, deliberative.
Explanations should include relevant features. Explanations should relate to the key features of the situation and the goals of the explainer and explainee.
Explanations should be framed to suit the audience. Explainers should fit the explanation to suit the explainee’s understanding of the topic and what it is they wish to gain from the explanation (their mental model and goals).
Explanations should be interactive. Explainers should involve explainees in the explanation.
Explanations should be (where necessary) actionable. Explainees should be given information that can be used to perform and/or improve future actions and behaviours.
No comments:
Post a Comment