By Peter Denning, Doron Drusinsky, and James Bret Michael
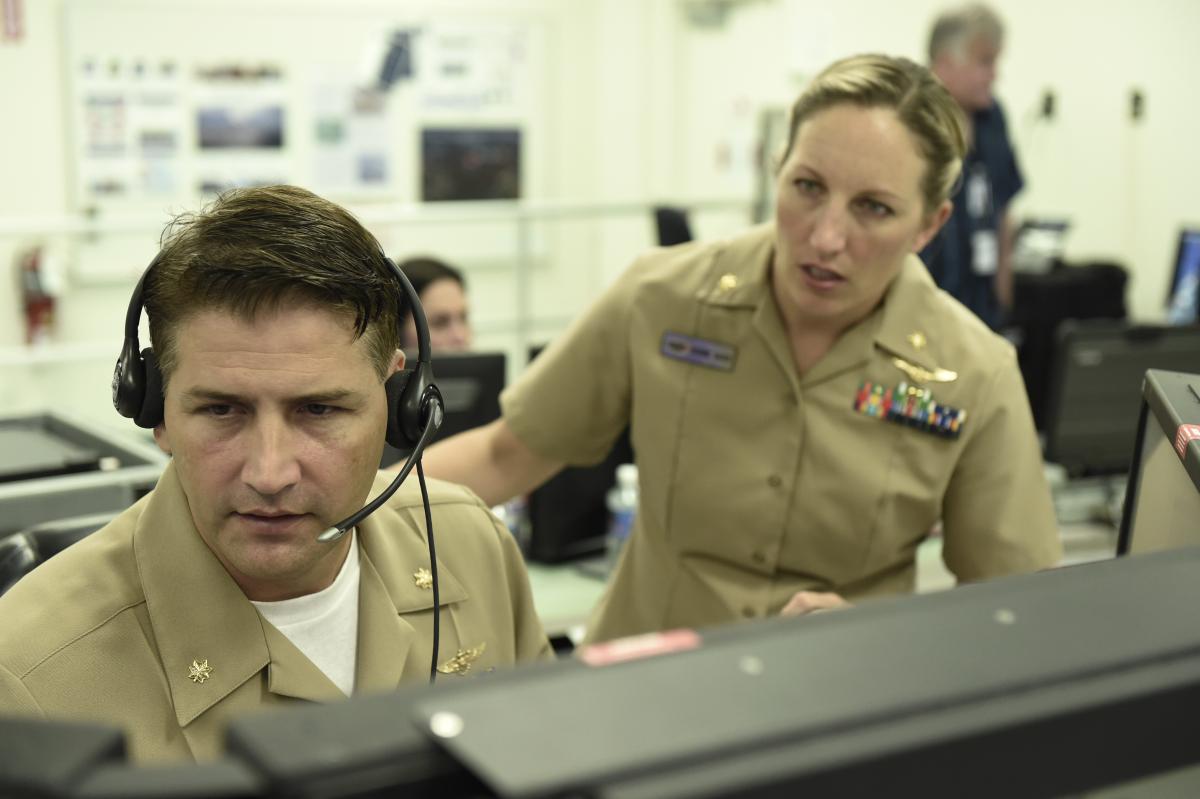
As military AI becomes more complex, the challenges increase for designers to guarantee reliable system operation and commanders to know when they can trust these systems. But there are vexing dilemmas designers face in confronting these challenges. Education can prepare warfighters for applying AI in their domains, resolving new dilemmas that arise, and accelerating the pace of adoption of new generations of AI systems. Knowledge of the dilemmas will help focus research to resolve them and will caution against rushing to implementations that are not mature enough to be trusted.
Dilemmas in Current Generation AI Systems
Most of the eye-popping recent advances in AI are the result of artificial neural networks. But military systems depend on other components as well, all of which affect their complexity, dependability, and trustworthiness. Core components of traditional military systems include sensors, weapons, command-and-control, battle management, and communications. AI adds three major new components to these systems:
• Learning machines, which learn about the operating environment and recommend preliminary actions.
• Reasoning machines, which apply the rules of engagement and other forms of human reasoning to outputs from learning machines and make final recommendations to the command-and-control and battle management system operators.
• Massive data sources, which are large-scale sensor networks and repositories of raw or partially processed data.
These new components will transform military systems in ways that are still difficult to discern.
Designers and users of these new systems have been confounded by dilemmas that undermine trust and slow implementation. These AI-extended systems are the context in which the dilemmas must be understood and resolved. The first step in dealing with dilemmas is recognizing them. The most prominent ones are discussed next.
Geopolitical Consequences of AI Advances
AI changes the balance of power by giving advantages to previously impoverished adversaries. A well-known example is a weapon system that locks onto a target and then pauses to wait for human confirmation to fire. While the human is deciding, an adversary’s fully automated system fires first and wins the battle. The AI gave an advantage to the adversary that turned our norm of human-in-the-loop into a vulnerability.
AI is just one of many technologies that are advancing at rates much faster than the Defense Department acquisition system delivery times of 10 to 15 years. Many AI technologies have a half-life of three years; they would be obsolete long before they could be delivered under the current system. The Defense Joint AI Center (JAIC) is searching for new ways to acquire AI technologies at market speeds, before other countries catch up and pass the United States by.
AI is opening new possibilities for old problems. An example is undersea communications. Radio-frequency signals are attenuated too rapidly in water to cover much distance. Acoustic signals travel farther but are hampered by voluminous ocean noises and are susceptible to eavesdropping. AI is enabling new forms of “acoustic steganography” that might make AI-decodable communication signals undistinguishable from ambient ocean sounds. Can these methods be used to successfully hide our own communications? Can they make undersea adversaries invisible? This could have major implications on the size of the underwater fleet as well as systems of communication with them. Would adversary submarines become less detectable with such technology?
Another example is the observability of the ocean. New generations of nanosatellite constellations, 5G networking, and machine learning will soon make the real-time data on positions and movements of all ships commercially available. These commercial services will employ technology and services previously available only to the government and military. How will the Navy implement traditional tactics such as hiding the fleet? What strategies can be developed instead?
Arms Control and Deterrence
Since World War II, countries have managed to avoid world wars in part by relying on confidence-building measures such as arms control treaties predicated in part on mutual deterrence.
That principle appears to break down in cyber warfare, in which AI will play a major role. A familiar plague in the cyber domain is the so-called zero-day attack, which is the surprise deployment of an attack against a vulnerability the other party does not know they have. Do such peculiarities of the cyber domain undermine deterrence? Can the principle of deterrence be extended into the cyber-AI domain? Are there other useful steps for deterrence?
Explainability and Fragility
The artificial neural network is the most common AI technology. These networks consist of many layers of artificial neurons connected by weighted links. These networks acquire their functions, not by programming, but by training from a massive number of examples. When training is done, the resulting matrix of inter-neuron connection weights comprises gigabytes of storage. What happens if the human operator wants to know why the network generated an unexpected or erroneous output? There is no way to know: The connection matrix is unintelligible. Explanations will have to come from the other components of AI-enabled systems.
Neural networks can be quite sensitive to small changes in their inputs. For example, changing a few pixels of a trained input image can cause an image classifier’s output to change significantly even though the human operator cannot see a difference in the image. A driverless vehicle that misinterprets a bird-dirtied stop sign as a yield sign can cause an accident.
Poor explainability and fragility are the opposite of robustness. They undermine the trust human operators are willing to place on neural networks in situations where their inputs have not been tested or when their outputs are surprising. For example, will a drone correctly distinguish friend from foe in a new combat zone? Can it explain to the human operator why it classified a target in a particular way?
Fragility also applies to seemingly small changes in the gathering of training data. Suppose two neural networks are each trained from different training sets taken as samples from a larger population. By all standard measures the two training sets are equivalently fair and unbiased representatives of the population. But in practice, the two networks may respond to the same input with different outputs. Statistically minor changes in the training data can result in major changes in the output.
Adversarial and Trustworthy AI
The domain of “adversarial AI” is burgeoning as militaries seek to defend against attacks that aim to disable their neural networks by confounding sensors with noise. The fragility of neural networks is a major vulnerability exploited by adversarial AI. Some pessimists worry that neural networks are so fragile that they cannot meet requirements for military-grade reliability. Will we be able to develop means for testing and evaluating systems to establish confidence in high-consequence applications?
Bias
Bias in training data can skew outputs. For example, an AI targeting system in a drone relies on training data that distinguishes images of humans from inanimate objects. The training data are drawn from many countries. The system may malfunction in real-time in a particular country where local dress customs may differ significantly from the average of the training data. The bias of the training data may be invisible to the people running the training algorithms and only becomes visible in the results when the neural network is presented with untrained inputs.
Reliable Training Data Is Expensive
Neural networks require large training sets. It is not unusual for an image recognizer to need 100 million labeled images for its training. Getting properly labeled data is time consuming and expensive. We saw a scenario in which $50 million in diagnostic physician fees would be required to gather 100 million labeled images to train a neural network to recognize cancerous colon polyps. This has encouraged use of open-source datasets obtained by paying cheap gig workers to spot suspicious features. How much trust can one place in a medical diagnosis system trained in such a way?
Robotic systems are even more problematic to train than image recognizers. Whereas the images to train a recognizer can be considered statistically independent, the images used by robots come in linked sequences that influence how the robots respond in different situations over time.
Deepfakes
Editing tools for images, videos, and audio tracks are being combined with AI tools to produce convincing fakes. They often cannot be distinguished from real images, videos, or audio without advanced equipment and forensic skills. How can we trust digital identifications when digitized forms of traditional identifications cannot be distinguished from fakes? How can we tell if an intelligence report is faked? The susceptibility of images to faking brings the art of deception to a whole new level when no one can tell what images or reports are real and authentic.
Human in the Loop
The military’s interest in AI to distinguish potential targets for attacks illustrates another dilemma: Should a drone be allowed to deploy its weapon without an explicit command from a human operator? If a weapon system uses AI to make decisions, should a human have the final say in whether a weapon is launched?
The argument for high levels of autonomy is that the pace of battle is likely to move at machine speeds. A completely autonomous incoming enemy swarm may be done with its work before our defending human operator can decide anything. The argument for keeping humans in the loop is that the fragility of AI systems can easily cause them to make mistakes no human would, precipitating or rapidly escalating an unwanted conflict. An example of a close call stemming from a misclassification by an electronic system was the Soviet nuclear attack false-alarm incident of 1983.
Not all human-in-the-loop systems are naturally replaceable by AI systems. For instance, in the 1980s the Navy fielded the expert system ZOG on an aircraft carrier. ZOG was intended to assist in the management of information and decision-making in combat situations, capturing all aspects of the ship’s tasks. Although ZOG operated correctly per its specifications, there was a serious mismatch between the assumptions used to design the system and the actual practice of ship operations. ZOG did not attain the desired level of teaming between humans and machines. Despite four decades’ experience building and fielding such systems, human-machine teaming continues to be an issue with expert systems, neural networks, and other Navy AI applications. This is not because human-machine teaming for decision-making is a bad idea, but rather because it is complex and demands more from the machine’s capabilities than can be implemented with current technology. ZOG and other projects show that contemporary AI works best for problems that enjoy mathematically well-defined domains of discourse and quality-of-outcome metrics. Fully automating certain human-based workflow processes, such as ship management, is not of this kind.
Cognitive overload is a human-factors aspect of the human-in-the-loop dilemma. Operators at sea can easily be overwhelmed by massive data. They are in the loop but impaired, because their brains cannot keep up with the data rate. AI can be combined with valued information at the right time (VIRT) models to deliver actionable information and screen out irrelevant information. Paradoxically, higher degrees of automation can actually hinder operators’ ability to maintain situational awareness, which exposes them to making poor decisions.
What AI Comes Next?
Although it is the center of attention in today’s AI systems, the neural network is a baby step on the ladder of learning machines that are likely to find their places in military AI systems (see Table 1). The neural network appears at Level 2 in a much larger hierarchy. It will not disappear, but it will be an adjunct of much more powerful reasoning systems designed to make sense of all recommendations in the context of battle. These systems will function in the presence of uncertainties, such as incomplete or conflicting information available during battle, from imperfections in the sensor networks, or from fragility in embedded neural networks. In addition to their advanced sense-making capabilities, these systems also will be able to reduce the need to train AI systems from external training sets, so fraught with difficulties.
Here are some examples that illustrate the possibilities available at the higher levels of AI machines. At level 3, in the 1980s, NASA researchers demonstrated AUTOCLASS, an AI system that took raw data from an infrared sky survey and, with no input from astronomers, accurately reproduced the classes of objects already identified by astronomers. AUTOCLASS demonstrated that a classifier system can self-train from its input data using the statistical method known as Bayesian learning.
At level 4, reinforcement learning pits two or more machines against each other to learn how to excel at a game, without knowledge of how humans played the game in the past. DeepMind’s AlphaZero technology, debuted in 2017, learned to play grandmaster chess in four hours with only the rules of chess but no data about past chess games. Military leaders are interested in whether this technology could be extended to wargames and battle management.
At Level 5, human-machine teaming allows combinations of humans and machines to coordinate to produce a result that neither could do alone. DARPA’s AI Next campaign envisions exactly this. For example, a human operator teamed with a swarm of autonomous drones might defend a ship against an incoming drone swarm attack.
These systems will surely be here in a few years and will go well beyond the capabilities of systems centered on neural networks. Equally surely, they will produce new dilemmas.
Responding to the Dilemmas
The dilemmas signal that neural network technology has limits in military utility. Resolving the dilemmas rests on a two-dimensional strategy. One dimension is investing in research that sheds light on the questions raised by the dilemmas and seeks solutions tailored to individual warfighting domains. The other is investing in the intellectual capacity of warfighters, acquisition professionals, and other stakeholders. A person educated in AI technology is less likely to be disrupted or overwhelmed by the appearance of a new dilemma.
The JAIC has adopted a plan with these two dimensions. It will support research that solves important operational problems and translates rapidly into use. It also is launching an education initiative that begins with an “AI for all” online course and will eventually develop AI curricula tailored to DoD workforce career paths.
The AI dilemmas are an early sign of disruption wrought by AI technologies. None is easily resolved. Most originate with today’s artificial neural networks, a technology of many imperfections. Future AI systems will overcome these imperfections by embedding neural networks into more complex AI systems that use reasoning, self-learning, reinforcement learning, and teaming. AI education, along with other measures such as acquisition reform, will enable the defense workforce to use these systems effectively and field new apps and tools rapidly—a civilian and military workforce capable of proactively identifying and resolving their dilemmas.
No comments:
Post a Comment