BY AARON BOYD
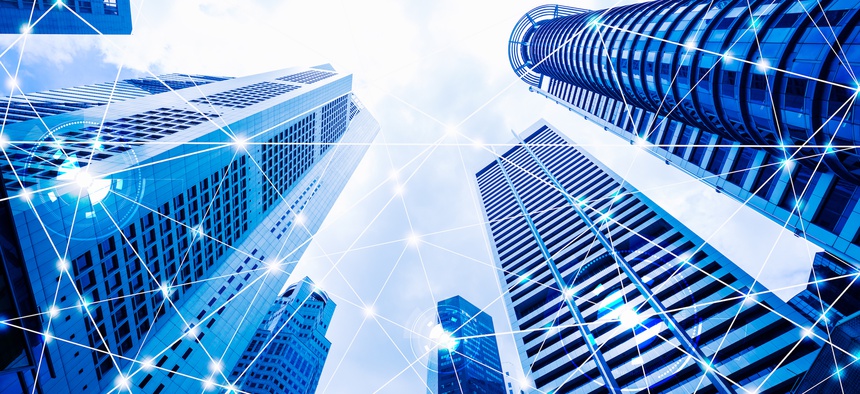
The Defense Advanced Research Projects Agency, or DARPA, has been instrumental in many of the most important breakthroughs in modern technology—from the first computer networks to early AI research.
“DARPA-funded R&D enabled some of the first successes in AI, such as expert systems and search, and more recently has advanced machine learning algorithms and hardware,” according to a notice for an upcoming opportunity.
The special notice cites the agency’s past efforts in AI research, including the “first wave”—rule-based AI—and “second wave”—statistical learning-based.
“DARPA is now interested in researching and developing ‘third wave’ AI theory and applications that address the limitations of first and second wave technologies,” the notice states.
To facilitate its AI research, DARPA created the Artificial Intelligence Exploration, or AIE, program in 2018 to house various efforts on “very high-risk, high-reward topics … with the goal of determining feasibility and clarifying whether the area is ready for increased investment.”
The special notice posted Wednesday announced an upcoming opportunity to work on In Pixel Intelligent Processing, or IP2, as a means of increasing accuracy and usability of video image recognition algorithms, particularly at the edge where sensors often don’t have access to enough power to process complex workloads.
“The number of parameters and memory requirement for [state-of-the-art] AI algorithms typically is proportional to the input dimensionality and scales exponentially with the accuracy requirement,” the special notice states. “To move beyond this paradigm, IP2 will seek to solve two key elements required to embed AI at the sensor edge: data complexity and implementation of accurate, low-latency, low size, weight and power AI algorithms.”
For the first part of the effort, DARPA researchers and partners will look at reducing data complexity by focusing neural network processing on individual pixels, “reducing dimensionality locally and thereby increasing the sparsity of high-dimensional video data,” the notice states. “This ‘curated’ datastream will enable more efficient back end processing without any loss of accuracy.”
That algorithm will pull out only the most “salient information” to transfer to a backend “closed-loop, task-oriented” recurrent neural network algorithm, which itself will be streamlined to limit power consumption.
“By immediately moving the data stream to sparse feature representation, reduced complexity [neural networks] will train to high accuracy while reducing overall compute operations by 10x,” DARPA officials said.
The resulting AI solution will be tested on a UC Berkeley self-driving vehicle dataset that features a host of challenges for computer vision, including “geographic, environmental, and weather diversity, intentional occlusions and a large number of classification tasks” that are “ideal for demonstrating 3rd-wave functionality for future large format embedded sensors.
No comments:
Post a Comment