By ZIGFRIED HAMPEL-ARIAS and JOHN SPEED MEYERS
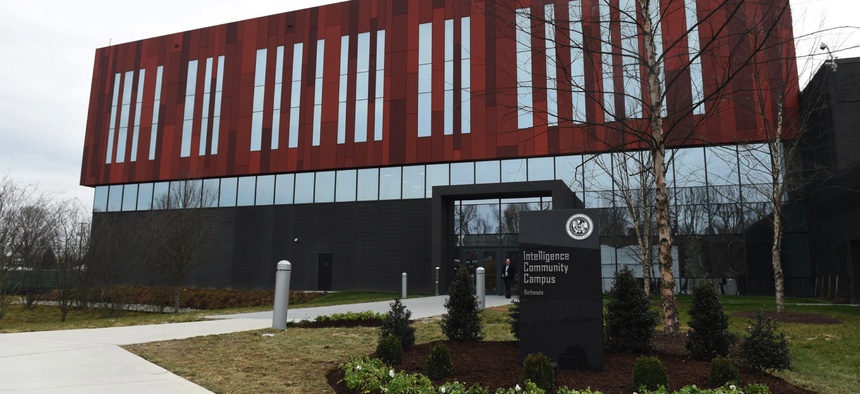
When we talk about AI’s prospective value in intelligence work, we are generally talking about the specific field of deep learning, a term that refers to multi-layer neural network machine learning techniques. Deep learning tools have made tremendous progress in fields such as image recognition, speech recognition, and language translation. But there are limits to its abilities.
Deep learning excels at “tasks that consist of mapping an input vector to an output vector and that are easy for a person to do rapidly,” wrote three of the field’s leading lights — Apple’s Ian Goodfellow and University of Montreal professors Yoshua Bengio and Aaron Courville — in their 2016 textbook Deep Learning. “Other tasks, that cannot be described as associating one vector to another, or that are difficult enough that a person would require time to think and reflect in order to accomplish the task, remain beyond the scope of deep learning for now.”
To recast these answers in simpler terms, these scholars are suggesting that modern AI can achieve extraordinary performance on what might be called “thinking fast” tasks but not on “thinking slow” tasks, to trade on the memorable terminology of Daniel Kahneman’s Thinking, Fast and Slow. “Thinking fast” tasks, for this essay, refer to tasks that involve a human or machine quickly and intuitively associating an input with an output, like spotting and recognizing planes. “Thinking slow” tasks are deliberate and do not require matching an input with an output, like determining the wisdom of purchasing a particular satellite.
A quintessential thinking-fast task is object detection in imagery intelligence. A human analyst can visually scan images for objects, such as planes or buildings. Deep learning computer vision techniques, including object detection within geospatial imagery, can also scan images for objects, aiding and indeed accelerating the processing of raw intelligence data. The body of geospatial machine learning research produced by the SpaceNet collaboration and the Defense Innovation Unit’s xView challenges makes this abundantly clear.
While SpaceNet’s work focuses on foundational mapping (e.g., recognizing building footprints), the research has implications for tasks traditionally associated with imagery intelligence. Just as a human would scan for wings and fuselages, modern deep learning models can perform an analogous task, detecting components of a plane and recognizing plane types. Of course, these geospatial models, like all models, are flawed, and care must be taken related to machine learning with off-nadir images (satellite images captured from an oblique angle), inadequacies in the data labeling process, and the need to customize models for specific tasks.
Other think-fast tasks related to collection, processing, and analysis may benefit from machine learning such as speech-to-text transcription, including identifying human speech in noisy environments and cross-language translation. Collection efforts can also take advantage of machine learning on “edge devices,” computer-speak for low-power, low-bandwidth devices operating in remote locations.
What can’t AI do for the IC?
Modern deep learning isn’t very good at deliberative thinking. It just doesn’t think slow, at least not yet. This deficiency means that those steps of the intelligence cycle that most require abstract deliberation—planning, communicating, and evaluating—will present serious machine learning difficulties.
Consider a machine learning model that can help intelligence analysts detect and categorize planes: determining whether to operate that model, how to communicate its results, and how to evaluate this model’s contribution are effectively impossible within the modern deep learning framework. These are not intuitive, associative tasks; we expect the intellectual dragons here to slay even the most capable machine learning practitioners. Even the intelligence analysis stage, which we categorized as part of “thinking fast,” contains higher-level questions beyond the purview of machine learning. Does a buildup of planes at certain bases indicate a surprise attack? You’d do better consulting works such as the RAND intelligence historian Roberta Wohlstetter’s Pearl Harbor: Warning and Decision or Columbia professor Richard Bett’s Surprise Attack, writings that will likely make you question that question itself.
Even when applied to thinking fast tasks, machine learning models have important weaknesses. All bets are off when interpreting models for which the training data and the real-world data diverge. For example, if a plane detection model was trained only with imagery of commercial airliners, then it cannot classify a MiG-29. The model was not built to provide such an answer. Similar caution also should be applied to models for detecting rare events, an unfortunate limitation given that intelligence work often involves rare events.
Evaluating intelligence activities will, our theory predicts, also be particularly difficult for machine learning. This function is the antithesis of the think-fast deep learning approach because it involves abstract, deliberative judgment. This is why you don’t see thinkers and writers interested in assessing the performance of the intelligence community relying on machine learning-based analysis to make their case. You want seasoned intelligence analysts doing this analysis, not Siri.
What Does This All Mean?
Most importantly, will robot overlords take over the middle of the intelligence cycle? We doubt it, or at least don’t know of compelling evidence to that effect.
Former intelligence analyst Zachary Tyson quotes venture capitalist Kai-Fu Lee: “Much of today’s white-collar workforce is paid to take in and process information, and then make recommendations based on that information — which is precisely what AI algorithms do best.” Tyson thinks that it is therefore doubtful that the intelligence analyst will remain central to the connection between intelligence and policy. Our theory, respectfully, disagrees.
First, Lee’s vague generality rides roughshod over what white-collar professionals do in general and what intelligence professionals do specifically. The intelligence community does take in and process information, but it also helps determine what information to take in (planning), helps communicate the information (dissemination) and helps compare the risk and usefulness of the endeavor (evaluation). Second, it’s not clear that “making recommendations” is what AI does best, despite Lee’s claim. AI might recommend the most appropriate GIF to you, but it’s highly doubtful that AI can prioritize the most important intelligence collection requirements. Third, it’s not clear that machine learning-powered intelligence analysis reduces the demand for analysts, or at least this claim deserves study and reflection, of the thinking-slow variety. Indeed, with current techniques, you still need analysts to train and evaluate AI models. Fourth, the output of machine learning algorithms applied to raw intelligence data is better thought of as intelligence foundations, not finished analysis;such analysis will continue to be the purview of skilled analysts.
What would be most useful to this debate is a clear, rigorous theory of the tasks at which modern machine learning excels and the tasks at which it fails. The theory we borrow from Goodfellow and his co-authors is only a few sentences in a book otherwise filled with linear algebra, deep learning methodology, and future research directions. A useful research contribution would be a meta-analysis that can improve upon this theory, which we have tried to apply to the intelligence community.
We realize that we are in murky waters and so expect lots of readers will disagree. We look forward to counter-arguments, especially any written with GPT-3. We should note that we expect your argument to be of the thinking-slow variety given that your review is really an evaluation activity. If it is, and you find machine learning techniques unhelpful in responding to our undoubtedly flawed argument, please ask yourself why. To thinking slow!
No comments:
Post a Comment