Alex MacCalman, Jeff Grubb, Joe Register and Mike McGuire
Introduction
Recent technological, socio-economic, and geopolitical trends, coupled with the reemergence of great power competition, complicate the future environment in which U.S. Special Operations Forces (SOF) must operate. SOF professionals will need to operate not only across traditional physical domains such as land, air, and sea but also in the virtual and cognitive domains. In particular, achieving cognitive dominance over adversaries will be essential to the success of future SOF missions.
Future innovations require a change in how the U.S. seeks military advantage through technology. Since the end of WWII, the U.S. relied on the development of advanced military-specific equipment, such as nuclear weapons, precision guided munitions, and stealth technology to deter or defeat adversaries. These technologies generally targeted physical objectives with kinetic effects and were, at least initially, beyond the capabilities of adversaries’ research and industrial bases to reproduce. In contrast, competition, as defined by the 2018 National Defense Strategy, will be explicitly multi-domain, with the U.S. and competitors simultaneously operating in not only physical domains, but virtual and cognitive domains as well.[i] Operating in the virtual domain involves seeking advantages in computer generated environments or cyberspace.[ii] Operating within the cognitive domain involves influencing the minds of potential competitors and populations.[iii] SOF must simultaneously dominate the physical, cognitive, and virtual domains in order to present multiple dilemmas that will achieve our desired outcome. Not only must we adopt our doctrine to operate in the multi-domain environment we must also advance technologies that will enable our newly defined doctrinal concepts.
Information processing technologies are proliferating at the lowest levels. Today, even mundane home appliances like TVs and refrigerators routinely carry an array of sensors and internet-connected embedded microprocessors. Every traffic signal light, cell phone and aircraft is a potential sensor platform and interface to the populous of an area—we live in a sea of data. Smart autonomous systems, mixed reality, Artificial Intelligence (AI) and Machine Learning (ML) algorithms, increased computational capabilities, and other emergent technologies will change the way we think and operate—especially as the digital universe grows exponentially with increased access to data that will fuel these algorithms.[iv]
We envision a next generation operator that can use all of the available data to aid decision making to reduce risk, manage uncertainty, and increase lethality. On the other hand, we also envision near-peer competitors who will attempt to do the same. Advancements in emergent technologies will be democratized and shared across the globe making it difficult to gain a competitive advantage based upon technology alone. Our increased use of networked technologies will provide new vulnerabilities for competitors to detect, track, and target. The data generated from the Internet of Things and the emergence of smart cities will require SOF to conceal or obscure their digital signatures while operating in these highly connected environments.
In the past, stealth, speed, and radio communications might have been enough to ensure SOF operators maintained an advantage. Now, radios have given way to cellphones, laptops, and networked edge computing devices that are already widely available to everyone. Tomorrow’s multi-domain competition will be won by those who are best able to extract timely, actionable information from the sea of data and provide it to an empowered operator postured to seize opportunities. Making sense of data at machine speed will be essential to allowing SOF professionals to retain their historic overmatch. Our advantage will come from our ability to find new access to data, more efficient means of analysis, and faster access to information at the decisive point. In order to embrace this future phenomenon, the U.S. Special Operations Command (USSOCOM) is pursuing the concept of the Hyper-Enabled Operator (HEO), to provide U.S. SOF with cognitive overmatch against future adversaries.
Cognitive Overmatch
The term “cognitive” is fairly broad and has been used in military contexts to mean many seemingly disparate things. For example, “cognitive maneuver” refers to actions that are intended to influence the thoughts, beliefs, and perceptions of a populace. In contrast, the Defense Advanced Research Projects Agency (DARPA) AugmentedCognition program sought to provide individual warfighters with adaptive automations to increase their situation awareness and reduce their mental workload.[v] The unifying feature of both of these uses of “cognition” is that they refer to a mental process by which information influences action.
John Boyd provided the most traditionally utilized theory that links cognitive dominance to military success.[vi] His prescription for defeating an adversary was to get inside the adversary’s Observe, Orient, Decide, Act (OODA) Loop. According to Boyd, competitor actions are the result of a process that starts with the observation of relevant data within a context of a situation. Competitors then synthesize the observed data with prior experience, doctrine, etc. to build their own understandings of the situation. Boyd referred to this building of situation awareness asorientation. Each competitor’s own picture of the world provides the basis on which that competitor decides on or selects from among available courses of action. The result of the subsequent action is then observed, providing the basis on which to update situation awareness. Figure 1 shows the “OODA Loop” model.

Boyd’s prescription for overmatch is to ensure that one’s own OODA loop yields superior actions faster than the adversary’s OODA Loop does. The competitor who predicts fastest and can take a more dominant action first will dictate the terms of the engagement. Fast, purposeful action creates a rapidly changing environment that renders the opponent’s current situational awareness inaccurate and further impedes their actions. Because of the democratization of emerging technologies, our competitors have the means to predict and act faster than we do. The future operating environment will not allow for time to deliberate within the OODA Loop. Our adversaries will leverage algorithms, compute power, and human-machine teaming to act fast. Therefore, we cannot afford to wait for complete information. Our competitive advantage must come from how quickly and effectively we can use the information we derive while balancing the decision speed and accuracy tradeoff. Culturally, we must learn to manage the risk of sacrificing accuracy to rapidly make decisions with incomplete information and act in anticipation of our adversaries rather than waiting to observe.
We define the term cognitive overmatch to mean the ability to dominate the situation by making informed decisions faster than the opponent. In order to achieve cognitive overmatch, we must leverage technologies to asymptotically drive the observe-orient-decide portion of the OODA Loop to zero. In the book “Hyperwar: Conflict and Competition in the AI Century” by Amir Husain et al., the authors describe how the fusion of distributed AI with autonomous systems will transform warfare in ways never seen before. The book states “The benefits enabled by a tightening of the OODA Loop are so significant and can enable such benefits in a battle that they simply cannot be overlooked or ignored.”[vii] In order to embrace the benefits of these emergent technologies, USSOCOM will accelerate SOF innovation with applied research focused on hyper-enabling the operator.
The Hyper-Enabled Operator
USSOCOM defines HEO as a SOF professional empowered by technologies that enhance the operator’s cognition at the edge by increasing situational awareness, reducing cognitive load, and accelerating decision making. In short, HEO hyper-enables the operator by providing technological aids to shorten the time within his OODA loop, thereby providing him with cognitive overmatch. The term “at the edge” refers to the austere, complex and denied environments the SOF professional will operate in while conducting Special Operation activities. The goal of HEO is to get the right information to the right person at the right time. The definition of an operator is any SOF professional that is operating at the edge; this may include a Civil Affairs professional, a helicopter door gunner, a cyber professional, a pilot, a boat driver, an assaulter, and many others. The term hyper implies that this will be a continuous and iterative process. USSOCOM hyper-enabled the SOF Operator recently through their early adoption and evolution of the Tactical Assault Kit (TAK). HEO will use the TAK-enabled Operator as the starting point and continually improve from that baseline over time.
Connecting SOF professionals with extended data processing and information visualization capabilities is already difficult today, especially when the operating environment is austere. As future population centers continue to grow, Megacities will become a prominent environment for SOF professionals, further complicating our ability to communicate and manage our signatures. Additionally, SOF professionals will operate in denied environments where our opponents will maintain relative freedom of action. While operating at the edge in these austere, complex, and denied environments, SOF professionals will not be able to rely on computing infrastructures that are located far to the rear. The practical implementation of HEO will be dependent on condensing the benefits of a networked infrastructure and implementing it in a smaller, more localized version.
The purpose of the HEO definition is to place the technological emphasis on the individual SOF operator that is performing SOF activities at the edge. This emphasis highlights the first SOF Truth, “Humans are more important than hardware,” and distinguishes the enabling technologies the operator will use at the edge. The HEO enabling technologies include data assets, adaptive and flexible sensors, scalable tactical communications, edge computing, embedded algorithms, and tailorable human-machine interfaces. These technologies will be integrated into architectures that will sense, monitor, transport, process, and analyze data to aggregate information that will inform tactical decisions at the edge.
Examples of HEO capabilities that can enhance cognition at the edge are real-time multi-spectral sensors fused to provide a higher probability of identification and characterization; live language translation that enhance communications; software defined radios that acquire digital signatures; computer vision and audio capabilities that cue other assets to accelerate decision making; biomedical and human performance tools combined with spatial arrangement of entities within the environment; social network visualizations and aggregate sentiment analyses that enhance contextual understanding of the human terrain[viii], and many others. It is important to note that these examples must be tested with carefully designed experiments to learn whether they actually have a positive impact towards accelerating a decision.
Accelerated, decentralized decisions made at the edge will provide the decisive advantages we need to compete against our near-peer competitors, especially against those that have centralized decision-making structures. Great power competition will rely less on attrition to achieve their objectives and more on undermining the opponent’s decision-making. In an article titled “Decision Maneuver: the Next Revolution in Military Affairs,” the authors describe a new type of maneuver that “combines disruptive new technologies in autonomy and artificial intelligence with new operational concepts for command and control.”[ix] New technologies combined with novel operational concepts will establish a new warfighting competition where the first mover to act at the edge will gain the advantage. Therefore, enabling the SOF operator with the right information at the right time with the right authorities while at the edge will be more important than sharing all the information available to everyone for a common operating picture.
A hyper-enabled operator will necessarily require a tightly interconnected network of sensors and systems supported by various services from a foundational infrastructure. Observing all relevant data and converting this data into an actionable information is more than we can expect for an operator—or the equipment he can reasonably carry—to do alone. HEO applied research will focus on integrating HEO enabling technologies to make best use of the information provided by the foundational infrastructure and sensors at the edge. To further delineate the HEO enabling technology pillars, we will use the concept of a system boundary to define the system of interest and its greater context.[x] Figure 2 shows the HEO system boundary that contains the operating environment along with the four enabling technology pillars used at the edge. Exterior to the system boundary are the elements that interact with the HEO system; these elements consist of the necessary enabling elements that are not at the edge, shown at the left of the figure, and capability areas related to HEO, shown at the right of the figure.
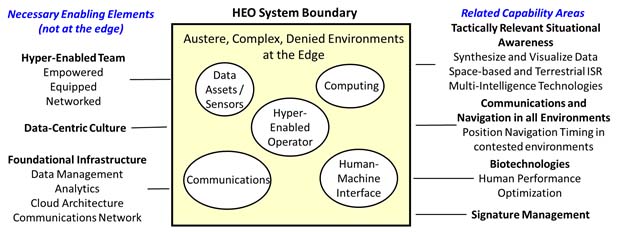
Figure 2. HEO System Boundary Diagram.
The line of demarcation of the HEO system boundary is arbitrary. Our intent is to delineate the HEO related research focus. For example, how an operator at the edge interfaces and controls terrestrial autonomous systems is within scope of the HEO research. However, research in improving terrestrial autonomous system effectiveness is a separate capability area that feeds the HEO. Subsequent sections will elaborate on each of the HEO enabling technology pillars. First, we will describe in more detail the necessary enabling elements needed to support a hyper-enabled operator that does not reside at the edge. These elements are the Hyper-Enabled Team concept, a data-centric culture, and a foundational infrastructure.
The Hyper-Enabled Team
The USSOCOM Future Operating Concept defines the hyper-enabled team as a combination of SOF professionals and partners that are empowered, equipped, and networked, to operate in the multi-domain environment of the physical, cognitive, and virtual domains.[xi] The empowered professional or team capitalizes on the mission command principles and philosophy to delegate the right authority coupled with a unified commander’s intent.[xii]Decisions must be made at the first level that has both the Commander’s Intent and the necessary information—and it will always be easier to push intent “down” than to pump information “up.” The competitive advantage of the HEO will come from SOF doctrine that exploits this ability to accelerate the decision cycle. The equipped professional or team employs technology to create decisive effects in all domains. These technologies encompass the entire portfolio of the SOF acquisition programs that are managed throughout various technology roadmaps.

Figure 3: The Hyper-Enabled Operator must be embedded within a Hyper-Enabled Team
The networked SOF professional has deep relationships with various elements inside and outside of the Government as well as our international partners. A second aspect of the network is the foundational infrastructure. The networked professional has a responsive Command and Control infrastructure that enhances situational awareness across all domains and partners. This allows us to aggregate knowledge across multiple information sources in various classification security domains to increase awareness. The proliferation of the Internet of Things, AI, and ML coupled with advanced networking infrastructures will enable the SOF professional to aggregate knowledge and be networked with various partners for a shared understanding. Figure 3 shows how the HEO concept is nested within the Hyper-Enabled Team.
Data-centric Culture
In order to truly enhance cognition at the edge, the SOF Enterprise must adopt a data-centric culture. Data can be in many structured, unstructured, and semi-structured forms including images, documents, audio and video files, marked-up languages, and tabular data frames. A data asset is something that brings value to the decision maker and must be managed and protected. This value is only realized when the right data is transformed into actionable information. The goal is not to generate more data; additional data does not necessarily increase the information available. HEO tools must allow us to focus our data collection in those places needed to reduce uncertainty, provide new insights, or improve predictive power. Increased access to the right data, transformed into actionable information will aggregate knowledge towards enhanced judgement. In order to effectively make use of data assets we first must embrace a data-centric culture by augmenting “gut” intuition with increased analytic insights fueled by an efficient data pipeline. Technology alone cannot deliver decision quality information. Expert knowledge in data analytics, data science, data engineering, software engineering, and other data-centric professions are needed to deliver insights and develop the embedded algorithms that will accelerate decision making at the edge. Pairing these data-centric professionals directly with SOF professionals in order to define problems, provide operational contexts, and deliver feedback will be critical to enabling a data-centric culture.
Foundational Infrastructure
The foundational infrastructure includes a data management strategy, analytics, a cloud architecture, and an advanced communication architecture. A disciplined data management strategy is essential to ensure that data is accessible, interoperable, trusted, secure, understandable, and usable. Coupled with the strategy is a data governance process that provides oversight on the data life-cycle management. The Data Management Body of Knowledge defines a recognized framework that describes the key knowledge areas that are necessary to govern a consistent strategy towards a data-centric culture.[xiii] These knowledge areas include data architecture, modeling and design, storage and operations, security, integration and interoperability, document and content management, reference and master data, warehousing and business intelligence, metadata, and quality. Instituting a strategy that implements these collective knowledge areas will facilitate the data pipeline needed to arrive at aggregated knowledge.
There are various forms of analytics that can enhance cognition. Descriptive analytics focus on exploring data for insights derived from existing data. These insights include the importance of outliers, hidden relationships, trends, and patterns. Diagnostic analytics attempts to explain what happened and generally involve performing regression techniques to explore the effects of a collection of independent variables (inputs) on a dependent variable (output). Predictive analytics builds data models that are trained with data to predict an outcome when given a new set of data. ML is a form of predictive analytics used to aid human decision making but implies a deep understanding of the problem. So deep - that the computer intelligently refines algorithmic parameters after it is presented with the problem many times, after many iterations of training. Other forms of predictive analytics include machine vision for object identification and characterization that rely heavily on labeled data. While ML algorithms will be a global commodity in many cases, relevant labeled data should be considered a critical military asset-- owned, protected and managed by the Government. Algorithms that aggregate knowledge from various data sources will come in the form of a predictive data model that can be embedded at the edge to enhance decision making. Prescriptive analytics is focused on using data to directly inform decisions and is best suited for planning, scheduling, and optimization of processes.
AI, at its core, is the ability to derive information from data as a human would. More explicitly, AI is the ability to leverage prior knowledge to extract implicit context from data and act or make recommendations upon that information. There are many forms of AI that would constitute combined usages of the previously mentioned analytic types as well as natural language processing, reinforcement learning, speech to text, text to speech, probabilistic models, expert systems, robotics, and many more. AI will better illuminate global conflict and transnational security issues including illicit threat networks, material supply chains, threat financing, organized crime, foreign fighter flow, social media trends. Common intelligence and operating pictures will no longer involve digital images of combined information but will serve as a digital workforce to advise the human and accelerate decision making.
The various forms of analytics described above have tremendous potential to speed the OODA loop at the edge. Table 1 lists each analytic type and where they can influence each element within the OODA loop.
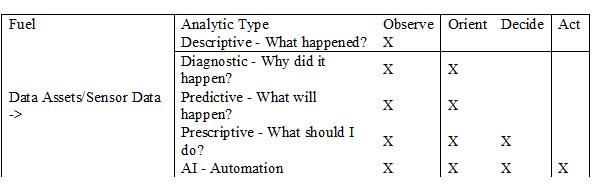
Table 1. Data analytic influence within the OODA loop.
The goal of the new paradigm shift towards leveraging analytics is to essentially force multiply the number of brains at the edge. This is achieved either through remotely assisting humans at the edge, operationalizing AI, or combinations thereof (human guided AI).
A cloud architecture is a key enabler to make use of data assets with an efficient data pipeline. USSOCOM is actively developing a cloud strategy for hybrid cloud development and application modernization using microservice architectures. The cloud strategy will enable things like Continuous Integration/Continuous Delivery (CI/CD) processes to improve experimental testing and speed capability deliveries. Additionally, the cloud will adopt microservices and orchestrate them with edge-based controllers. Cloud-based AI tools and processing power provide the critical backbone to train and deploy efficient models that can be used for inference further towards the edge. These microservices will be implemented using software containers and will run on devices worn by SOF operators and other platform assets to allow for redundant and distributed computing. The nature of the microservice architectures allows for rapid adaption to new hardware and rapid reconfiguration. The intent is to deploy a cloud environment that will perform all of this across unclassified and classified domains to maximize the effect.
The communications architecture is a tactical mission network that provides the data links needed to transport data. The operator must be able to seamlessly share information with other networked professionals within the Hyper-Enabled Team. This team needs expeditionary communication and position, navigation and timing capabilities that do not rely on satellites and function in austere locations, megacities, and denied areas. Near range communications between personal and machines will involve wireless area networks using either non-internet protocol (IP) messages or IP-based communication systems. Long-range communications are moving towards a cognitive approach where many modalities will be used in concert to get to an end result. In the future, data will be broken up and chunked by a cognitive router across Wi-Fi, cellular, satellite, and several different radio or free space optics and other non-RF techniques. As the communication paths degrade or change due to environment and distance the system should adapt and close the loop to provide continued service.
HEO Architectures
There will be many forms of HEO architectures that will be analogues to the Internet of Things architectures. Each HEO architecture will involve a variety of architectural designs decisions including sensor types, routing and protocols, networked communications, edge computing, cloud services, analytics, size, weight, and power constraints, and security. A HEO architecture will be a networked multi-tiered node topology. Physical examples of nodes are end user devices, sensors, radios, computing devices, routers, platforms, space assets, or cloud types. These nodes are connected via various types of data links forming a topology that constitutes a deployment of a HEO architecture. Nodes at the edge focus on senor data collection with some ability to control the device autonomously with limited analytics. Nodes at the next higher tiers are computing devices referred to as the Fog nodes that focus on data filtering, compression, and transformation with some amount of analytics.[xiv] These Fog nodes may be linked together to form mesh networks that balance processing, share data, increase resilience, and minimize the need to transport data to the cloud. The nodes at the highest tiers are generally in the cloud and focus on aggregating data and processing high end analytics to generate knowledge.
The purpose of the multi-tiered layers is to deal efficiently with the data that needs processing as it is aggregated across several nodes in the topology. The farther the data is transported away from the edge, the greater the insights that can be realized. Processing the data where it resides minimizes the need for transport. However, edge computing cannot match the processing power of the cloud. Size, weight, and power constraints limit the amount of processing we can do at the edge. The data link bandwidth constraints limit the amount of data that can be transported up the multi-tiered topology chain. Additionally, the higher the data is transported up the chain the more latency there is before the insights can be delivered back to the edge. Security is another aspect that further constrains the environment.
To bridge data from sensors to the cloud, we need smart routers and supporting IP-based protocols designed for efficiency. The role of the router is to secure, manage, and steer data. Edge routers orchestrate and monitor mesh networks to ensure quality data is transported; this will likely be in a software defined network tier that organizes and executes orchestration on sub-tiers. AI/ML algorithms will facilitate the opportunity to develop cognitive routers to monitor the environment and autonomously make data management and routing decisions with efficient, power-aware, low-latency protocols and compression algorithms. Different HEO use cases will have different data, processing, communication, and security needs and require unique node topology architectures to deploy the desired capability.
HEO Enabling Technologies
This section describes each of the enabling technology pillars highlighted within the HEO system boundary shown in Figure 2.
Data Assets / Sensors
Standoff sensors that identify and characterize individuals and objects in the environment based on physical, behavioral, physiological, electromagnetic, and other signatures will generate limitless data that must be managed, transported, and processed. We need architectures that fuse several sources of data at the edge that leverage computer vision, multi-spectral fusion, biometrics, and other telemetry systems to gain better insights within the multi-domain environment. We must also aggregate data across security domains to provide higher quality information from the analytical combinations of multi-sourced data.
Communications
Because we will operate in austere, complex, and denied environments, we must reduce the amount of data back-haul and improve long-haul communications with a low probability of interceptions and detection. Recently, we see communications within industry moving at a lighting pace with developments in new waveforms, custom application-specific silicon chips, compression techniques and software defined radios. Data rates have grown almost exponentially year after year for Wi-Fi and cellular data links. The recent advancements in coding schemes, timed transmission standards, antennas, and the coordinated use of multiple RF layers working in concert have all recently catapulted the speeds possible.[xv] New military radios now are starting to embrace some of these industry derived technologies and are including things like 4G modulation and multi-in multi out (MIMO). The challenge is to keep up. The SOF enterprise is now pressured to adopt these tools at a swift pace while navigating a minefield of potential security pitfalls. Finding and exploiting commercial advancements in communications while balancing the security concerns is key to rapidly developing SOF-peculiar solutions.
Computing
Computing involves both custom processing hardware and embedded software algorithms. These edge computing systems are limited by size, weight, and power constraints and therefore need clearly defined functionality with disciplined tradeoffs that will provide the required capability. Algorithms embedded in forward edge computing must dynamically analyze various sensors, adapt their digital signatures, and selectively transmit relevant information with the appropriate compression algorithms. We need algorithms that can extract features from sensor feeds, monitor changing data links conditions and adapt compression algorithms for various bandwidths. A key part to using these algorithms will be the new wave of custom hardware developed for edge-computing. Processors like tensor processing units (TPUs) operate now just as GPU with custom silicon chips for more efficient AI processing. As these developments happen, we must embrace the new hardware and use it for efficient edge processing.
Human-Machine Interfaces
The interfaces by which the operator accesses and interacts with information must be both flexible and intuitive. For information presentation, the interfaces should take advantage of how people naturally take in information. For example, directional hearing spatially segregates auditory input, not only enabling people to intuitively know where someone speaking is but to better attend to and track who said what. Replacing the current non-directional headphones with 3D audio systems may allow operators to better understand and attend to radio traffic. Likewise, appropriately implemented haptic devices could be used to provide spatially specific references and alerts, helping operators to maintain situation awareness. Providing the operator with voice or gesture control may provide an intuitive way to control and manipulate the available data.
The way information is presented to the operator must not interfere with critical perceptual tasks. Augmented reality systems could allow the operator to view an arbitrarily large virtual computer screen populated with multiple Intelligence, Surveillance, and Reconnaissance feeds, maps and text information. However, if that virtual screen blocks the operator’s view of the real world in critical situations, it would reduce his situational awareness. Such displays must be easily tailorable by the operator so that he can make use of their additional capabilities when they are useful and get rid of them when they are not.
In addition to digesting information, the human-machine interface technology pillar also includes the human’s ability to interact and control smart autonomous systems. The combined effects of AI, sensors, and autonomous unmanned aerial systems and how the SOF operator interfaces with these systems of systems is a critical research focus.
Experimental Process
In order to realize the potential of these emerging technologies that will hyper-enable the operator, we must perform disciplined experiments while fostering a culture of learning. Transformational innovative solutions start with ideas that solve problems and evolves into unsophisticated prototypes that are tested. By experimenting on ideas with prototypes we learn whether these proposed solutions solve a clearly defined problem and inform our future investments in technology. Additionally, experimentation allows us to illuminate the tradeoffs as earlier as possible so that the users can be involved with the critical design decisions throughout a system’s development life-cycle. Maximizing the learning potential as early as possible will ensure that we converge on viable solutions to solve problems. Figure 4 shows a six phased experimental process that integrates prototypes into experimental architectures to test ideas.
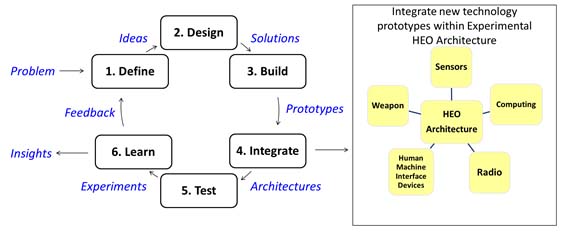
Figure 4. Experimental Process
The first phase involves clearly defining the problem while identifying future concepts and uses cases. Additionally, we must interview users, perform industry research and market intelligence to understand the technology space. These activities result in well-defined research questions and solution ideas with identified trade space variables. Once the define phase is complete, we arrive at a decision gate to decide whether to prototype an idea or not with clearly defined criteria. If the criteria are meet, we then design solutions, build prototypes, integrate them into experimental HEO architectures, and perform designed experiments to test ideas and learn. The experiments will result in either a positive or negative learning outcome. Positive outcomes will be scrutinized further to understand the idea’s core utility, assess the prototype performance, identify the technical limitations, and perform follow on experiments as needed. Negative outcomes will be celebrated as valuable learning outcomes of clearly designed experiments. The outputs of the experimental process ultimately inform the SOF Enterprise user community and future investment decisions. The potential insights that emerge from the process can be in many forms; they may increase knowledge on existing capability gaps, inform industry solicitations on SOF needs, derive key performance parameters and key system attributes threshold and objective levels, identify technology roadmap insertion points, provide technology readiness assessments, or refine basic research questions. The experimental process is not linear; at any point we may revert back or accelerate forward during the process. The ultimate goal of the process is to answer whether an idea is a viable solution to a problem.
To validate HEO ideas, we must develop testbeds with various architectural designs. There are many ways to deploy a HEO architecture that are specific to a chosen use case or scenario. Identifying these use cases along with clearly defined problems will enable us to test ideas that will hyper-enable the operator. Figure 2 highlights the HEO applied research focus. It is important to note that the interface between the foundational infrastructure and the HEO system boundary, shown in Figure 2, implies a critical coordination point between the HEO applied research focus and the acquisition management of ongoing programs. Insights derived from the HEO applied research will illuminate limitations, trade spaces, constraints, and identify opportunities and risks. These insights will help accelerate innovation by understanding early the implications of disruptive technology and how they viably can be delivered.
Conclusion
In order to maintain the competitive advantage against competitors, SOF must simultaneously dominate the physical, virtual, and cognitive domains in tomorrow’s future operating environment. The side that cognitively dominates the situation by making informed decisions faster will have the most influence in competition and will win in conflict. The Hyper-Enabled Team must quickly and effectively use information to predict and act while leveraging the decentralized flexibility of the SOF professional. We must adapt to get the right information, to the right person, at the right time to act decisively rather than emphasizing a common operating picture with all information for everyone. The HEO concept seeks to achieve this end by enhancing cognition at the edge and will continue to evolve as warfighter needs change and new technologies emerge. HEO is a vision that USSOCOM will continue to advance towards.
USSOCOM will achieve transformational innovations towards a hyper-enabled operator by clearly defining problems. Generally, solutions will constitute integrated system of systems composed of elements within each of the sensor, communication, computing, and human-machine interface enabling technology pillars. These solutions will leverage the empowered, networked, and equipped Hyper-Enabled Team, a data-centric culture, and a networked foundational infrastructure. Carefully designed experiments and agile processes that will rapidly test ideas with prototypes will foster the type of learning culture needed to inform whether we should refine, pivot, or kill an idea. The faster we can arrive at this type of learning the better we can “Accelerate SOF Innovations.”
No comments:
Post a Comment