SEAN GALLAGHER
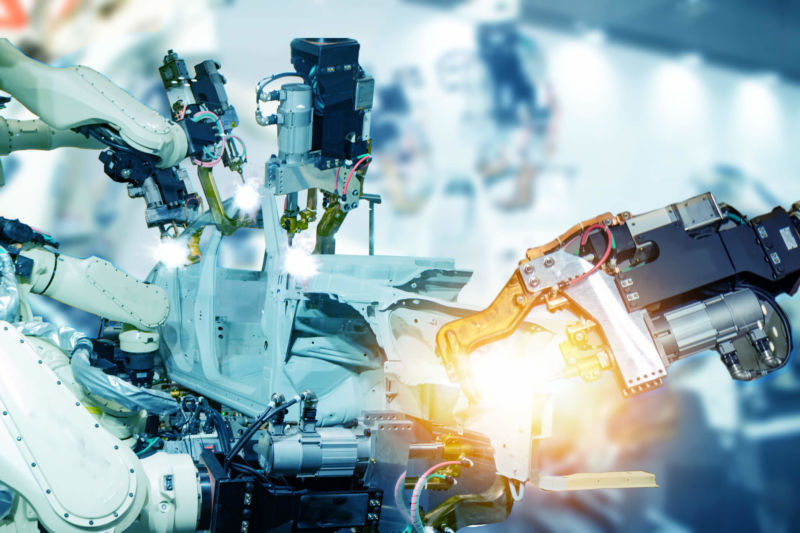
It's no surprise that the concept of "information about everything" is being aggressively applied to manufacturing contexts. Just as they transformed consumer goods, smart, cheap, sensor-laden devices paired with powerful analytics and algorithms have been changing the industrial world as well over the past decade. The "Internet of Things" has arrived on the factory floor with all the force of a giant electronic Kool-Aid Man exploding through a cinderblock wall.
Tagged as "Industry 4.0," (hey, at least it's better than "Internet of Things"), this fourth industrial revolution has been unfolding over the past decade with fits and starts—largely because of the massive cultural and structural differences between the information technology that fuels the change and the "operational technology" that has been at the heart of industrial automation for decades.
As with other marriages of technology and artificial intelligence (or at least the limited learning algorithms we're all currently calling "artificial intelligence"), the potential payoffs of Industry 4.0 are enormous. Companies are seeing more precise, higher quality manufacturing with lowered operational costs; less downtime because of predictive maintenance and intelligence in the supply chain; and fewer injuries on factory floors because of more adaptable equipment. And outside of the factory, other industries could benefit from having a nervous system of sensors, analytics to process "lakes" of data, and just-in-time responses to emergent issues—aviation, energy, logistics, and many other businesses that rely on reliable, predictable things could also get a boost.
But the new way comes with significant challenges, not the least of which are the security and resilience of the networked nervous systems stitching all this new magic together. When human safety is on the line—both the safety of workers and people who live in proximity to industrial sites—those concerns can't be as easily set aside as mobile application updates or operating system patches.
And then there's always that whole "robots are stealing our jobs" thing. (The truth is much more complicated—and we'll touch on it later this week.)
Sensors and sensibility
The term "Industry 4.0" was coined by Acatech (the German government's academy of engineering sciences) in a 2011 national roadmap for use of embedded systems technology. Intended as a way to describe industrial "digitization," the term was applied to mark the shift away from simple automation with largely stand-alone industrial robots toward networked "cyber-physical systems"—information-based orchestration between systems and the humans working with them, based on a variety of sensor and human inputs.
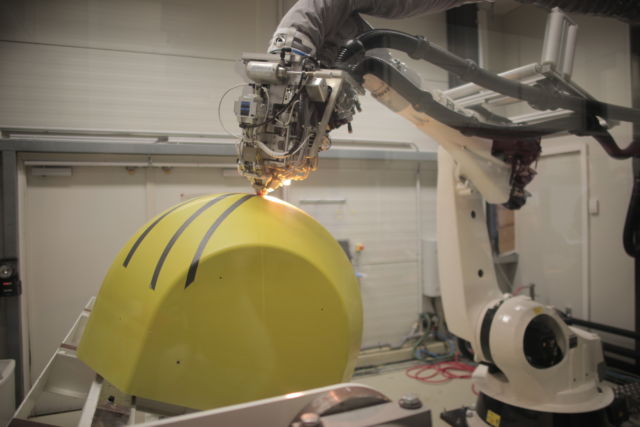
As a promotional document for the roadmap from the German Federal Ministry of Education and Research stated, "Machines that communicate with each other, inform each other about defects in the production process, identify and re-order scarce material inventories... this is the vision behind Industry 4.0."
In the Industry 4.0 future, smart factories using additive manufacturing—such as 3D printing through selective laser sintering—and other computer-driven manufacturing systems are able to adaptively manufacture parts on demand, direct from digital designs. Sensors keep track of needed components and order them based on patterns of demand and other algorithmic decision trees, taking "just-in-time" manufacturing to a new level of optimization. Optical sensors and machine-learning-driven systems monitor the quality of components with more consistency and accuracy than potentially tired and bored humans on the product line. Industrial robots work in synchronization with the humans handling more delicate tasks—or replace them entirely. Entire supply chains can pivot with the introduction of new products, changes in consumption, and economic fluctuation. And the machines can tell humans when the machines need to be fixed before they even break or tell people better ways to organize the line—all because of artificial intelligence processing the massive amounts of data generated by the manufacturing process.
That vision has driven a 1.15 billion Euro (approximately $1.3 billion) European Union effort called the European Factories of the Future Research Association. Similar "factory of the future" efforts have been funded by the US government—in particular, by the Department of Defense, which sees the technology as key to the defense industrial base.
The Defense Advanced Research Projects Agency (DARPA) has used research programs such as the Adaptive Vehicle Make project to seed development of advanced, information-integrated manufacturing projects and continues to look at Industry 4.0-enabling technologies such as effective human-machine teaming (the ability of machines to adapt to and work side by side with humans as partners rather than as tools) and smart supply chain systems based on artificial intelligence technology—an effort called LogX. Researchers at MITRE Corporation's Human-Machine Social Systems (HMSS) Lab have also been working on ways to improve how robotic systems interact with humans.
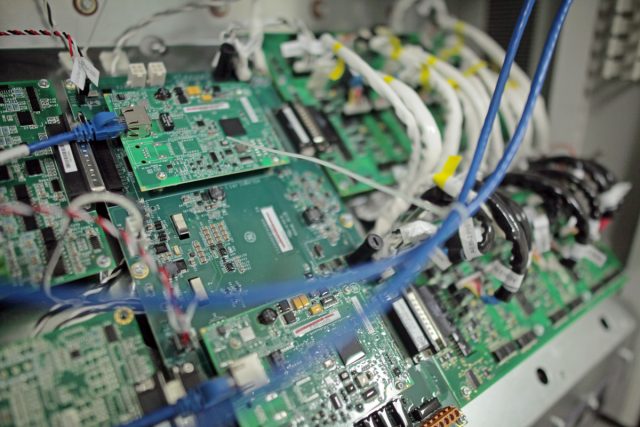
Greg Russ
As part of that work, MITRE has partnered with several robotics startups—including American Robotics, which has developed a fully automated drone system for precision agriculture. Called Scout, the system is an autonomous, weather-proofed unit that sits adjacent to fields. All a farmer has to do is program in drone flight times, and the AI handles drone flight planning and managing the flight itself, as well as the collection and processing of imagery and data, uploading everything to the cloud as it goes.
That level of autonomy allows farmers to simply look at data about crop health and other metrics on their personal devices, and then act upon that data—selectively applying pesticides, herbicides, or additional fertilizers if necessary. With some more machine learning juice, those are tasks that could eventually be handed off to other drones or robotic farming equipment once patterns and rules of their use are established.
Scout mirrors how human-machine teaming could work in the factory—with autonomous machines passing data to humans via augmented vision or other displays, letting humans make decisions based on their skills and knowledge of the domain, and then having humans and machines act upon the required tasks together. But that level of integration is still in its infancy.
Every sensor tells a story
One place where an embryonic form of human-machine teaming already takes place is in the world of retail: Walmart uses robots to scan store shelves for stock levels and has automated truck unloading (via a system called the "Fast Unloader") at many stores—using sensors and conveyor belts to sort shipments onto stocking carts. And robotic systems have already taken over the role of warehouse "picking" at Amazon, working with humans to retrieve and ship purchases.
Conversely, an element of Industry 4.0 that has evolved past the embryonic stage is the use of sensor data to drive plant operations—especially for the task of predictive maintenance. Unexpected equipment downtime is the bane of all industries, especially when the failure of a relatively minor part leads to the total failure of an expensive asset.
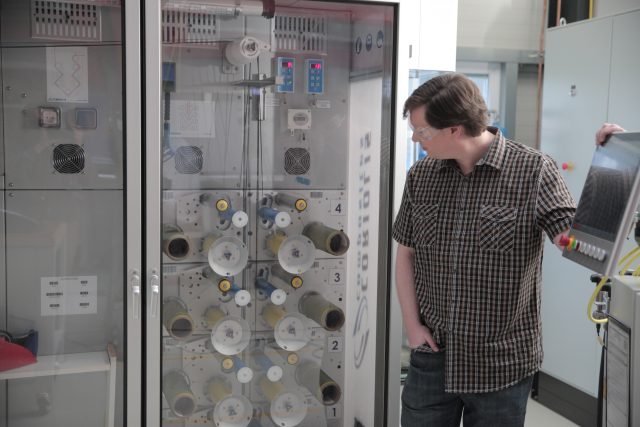
By some estimates, about 80 percent of the time currently spent on industrial maintenance is purely reactive—time spent fixing things that broke. And nearly half of unscheduled downtime in industrial systems is the result of equipment failures, often with equipment late in its life cycle. Being able to predict failures and plan maintenance or replacement of hardware when it will have less impact on operations is the Holy Grail of plant operators.
It's also a goal that industry has been chasing for a very long time. The concept of computerized maintenance management systems (CMMS) has been around in some form since the 1960s, when early implementations were built around mainframes. But CMMS has almost always been a heavily manual process, relying on maintenance reports and data collected and fed into computers by humans—not capturing the full breadth and depth of sensor data being generated by increasingly instrumented (and expensive) industrial systems.
Doing something with that data to predict and prevent system failures has gotten increasingly important. As explained by MathWorks' Industry Manager Philipp Wallner, the mounting urgency is due to "[T]he growing complexity that we're seeing with electronic components in assets and devices, and the growing amount of software in them." And as industrial systems provide more data about their operations on the plant floor or in the field, that data needs to be processed to be useful to the operator—not just for predicting when maintenance needs to occur, but to optimize the way equipment is operated.
No comments:
Post a Comment