By SYDNEY J. FREEDBERG JR. and PAUL MCLEARY
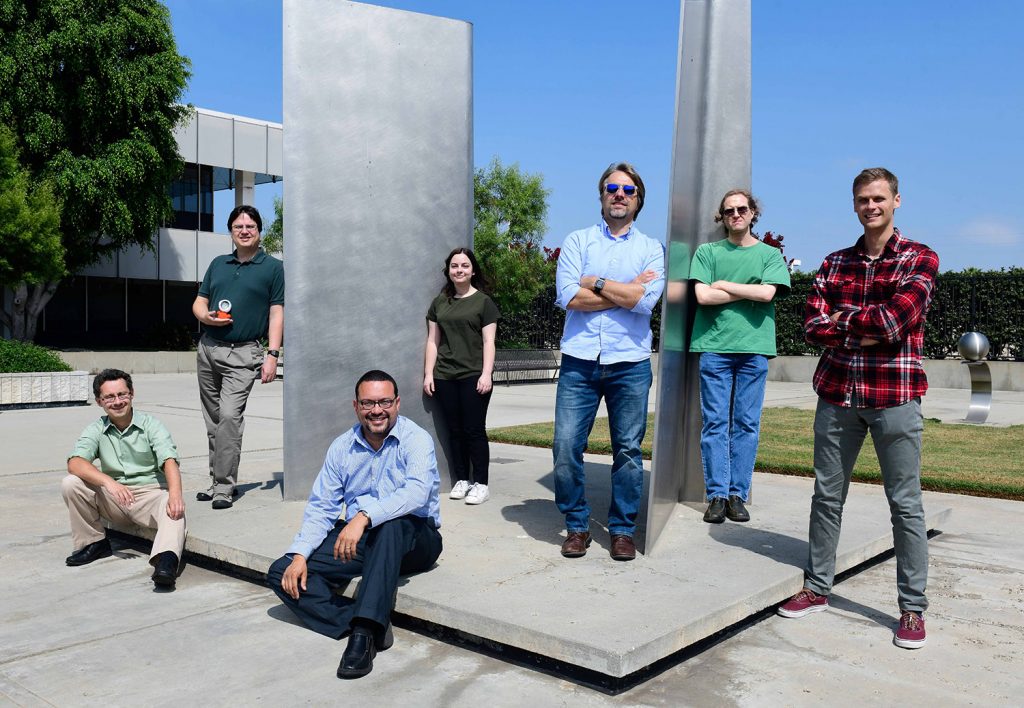
Steven Walker
One near-term example is DARPA’s Blackjackprogram, Walker said. Blackjack will build a network of small, affordable surveillance satellites in low earth orbit that can communicate with each other and coordinate their operations without constant human control. But, like most DARPA projects, Blackjack is just a “demonstration” that the technology can work, not an operational system.
DARPA’s specialty is long-term gambles, high risk and high reward, and Walker himself acknowledged that the AI technology of today still faces severe limits. So how can we cut through the hype to see what artificial intelligence and machine learning can really do for the US military in the near future?
One answer, in microcosm, might be a much more modest $100,000 prize that the Army’s Rapid Capabilities Office – whose mission is meeting urgent frontline needs – recently awarded to the gloriously named Team Platypus. The near-term payoff for military AI isn’t replacing human soldiers in the physical world, but empowering them to understand the world of radio waves. That’s an invisible battlefield which Russia’spowerful electronic warfare corps is poised to dominate in a future war, unless the US can catch up.
Humans Vs. Computers: Who Wins Where?
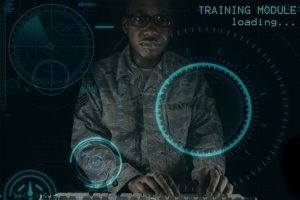
The whole military is keenly interested in artificial intelligence to sort through hundreds of terabytes of surveillance imagery and video collected by satellites and drones, sorting terrorists from civilians and legitimate targets from, say, hospitals. That’s the purpose of the infamous Project Maven, which Google pulled out of on ethical grounds (while secretly helping China censor Google Search). But AI object recognition is another nascent science. Former DARPA director Arati Prabhakar liked to show reporters an image of a baby playing with a toothbrush that a cutting-edge AI had labeled “a young boy is holding a baseball bat.”
But there are some areas where artificial intelligence can already outdo humans. Chess and Go are just the highest-profile cases. These phenomena tend to involve two things: There’s a staggering number of potential outcomes, but each outcome is rigidly defined, with no ambiguity. That makes them overwhelming for human intuition but amenable to the crisp either-or of binary code.
Andres Vila
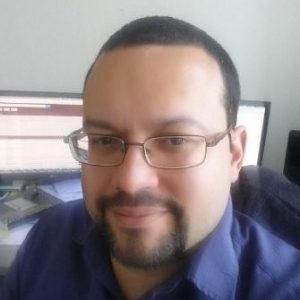
“Humans are really good at some of these problems, like image recognition,” said Andres Vila, the US-educated, Italo-Colombian head of the triumphant Team Platypus. Compared to machines, he told me, “humans still have the advantage at picking out chairs and cats (from other objects), but how many humans do you need to process a bunch of data?”
When it comes to radio communications, however, machines have the advantage even for analyzing a single signal, let alone large amounts of data. Instead of comparing what you see on a screen to a booklet of known signals, flipping pages until you find one that looks right, the software can check the precise figures against millions of potential matches.
“For this kind of coms problem, machine probably is better than human,” Vila said in an interview. “We can’t sit there and look at the raw signals that output and classify them correctly. There is just no way our own eyes could do it.”
Into the Matrix
Radio waves are vital to a modern military, carrying everything from verbal orders to targeting data, from search radar to electronic jamming. But unlike the signal flags or marching drums of past battlefields, radio is something soldiers can neither see or hear.
It’d be cool if we could train humans to see radio, chuckled Rob Monto, director of emerging technologies for the Army Rapid Capabilities Office: “It’d be like the Matrix.” But until someone develops cyborg eyes, we need machines to see for us – and the smarter the machine, the better.
The Army disbanded its Combat Electronic Warfare Intelligence (CEWI) units, like the one shown here, after the Cold War.
Traditionally, human specialists in signals intelligence and electronic warfare stared at screens displaying such data as the strength, direction, and modulation of radio signals. Then they compared the readings to a catalog of known enemy systems, each of which a unique way of transmitting based on its hardware. Back then, changing a radio’s or radar’s emissions required physically rewiring it.
But modern software-defined radio can emit a wide variety of signals from the same piece of equipment, and you can change those signals as easily as uploading new software. What’s more, because this technology has become so cheap and compact, the military aren’t the only people running around with radios anymore. You probably have one in your pocket: It’s called a cell phone. More radio emissions are coming from wireless networks, digitally controlled car engines, and even baby monitors.
So not only can the enemy easily change the signals their systems emit: They can also hide those changing signals amidst a zoo of civilian signals that are themselves constantly changing. If old-school electronic warfare was like finding a needle in a haystack – not impossible if you use a magnet – modern EW is like finding a particular needle in a needle factory…in the middle of a tornado.
It doesn’t matter how many humans you have staring at screens: They won’t be able to keep up. “The amount of brain matter you’d need to apply is not just practical,” Monto told me. You need something that thinks not only faster than an organic brain, but differently: an artificial intelligence.
Russian Krasukha-2 radar jamming system, reportedly deployed in Syria
The Challenge
The Army created its Rapid Capabilities Office to bypass the usual, laborious procurement system, which tends to take that soldiers are only issued information technology long after the commercial sector has made it obsolete. In this case, instead of holding a traditional competition for a government contract, the RCO took copied DARPA and held a “challenge,” offering cash prizes to whatever corporate or academic team could best perform a given task.
Seven autonomous cybersecurity systems face off for the DARPA Cyber Grand Challenge in 2016. DARPA has pioneered using competitions instead of traditional contracts.
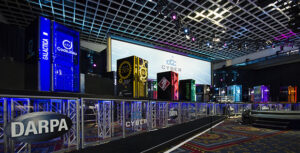
More than 150 teams participated to some degree, of which 49 made it to the actual competition. All of them paid their own way. The Army only had to shell out
$20,000 for the third place finisher, the private sector team THUNDERING PANDA (allcaps is mandatory) from Motorola;
$30,00 for second place TeamAU, a group of individual scientists from Australia; and
$100,000 for the champion, Team Platypus from the nonprofit, federally funded Aerospace Corporation.
Vila had joined Aerospace a few years ago specifically to work on this problem, realizing that all the machine learning technology Google and Facebook had developed for image recognition would translate well to radio signal classification. His team of eight engineers from multiple countries named itself in honor of the platypus, which can sense the electromagnetic fields of its prey.
The platypus uses electromagnetic fields to sense and hunt down its prey. (Meera Pate, Reed College, “Platypus Electroreception”)
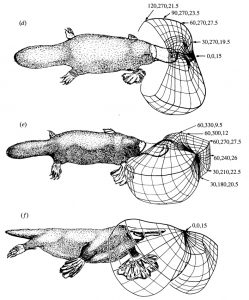
So what comes next? The Army is looking at two options, the RCO’s Rob Monto told me. One is to invite the high-performing teams from this challenge back for a second round, with a more sophisticated dataset and more challenging classification tasks, so they can further refine their software. The other is to get the software as-is into the hands of Army electronic warfare soldiers right away, so they can try it out in field conditions and give feedback. Ultimately the Army needs to do both software refinement and soldier testing, preferably in multiple rounds, but which comes next is still being studied.
At some point, whoever gets the actual contract will also need security clearance to look at classified data. The datasets used so far are unclassified, not secret information on potential enemy’s radios, radars, and jammers. Now, sorting through civilian signals is already useful in itself, Monto said: By telling soldiers what’s a cellphone, what’s a coffee shop wifi, and so on, it can let them focus their human brainpower on the anomalies that might be enemy activity. But ultimately the Army wants the software to help classify military signals as well.
Pop culture’s answer to “can we trust artificial intelligence?” (Courtesy Warner Brothers)
Ambiguity and Trust
Even cutting-edge artificial intelligence has its limits, Team Platypus’s Vila told me. Most obviously, it still can’t cope with novelty and ambiguity the way a human can. Machine learning is only as good as the data it was trained on. If you put AI up against “something absolutely brand new that we have never seen before… and couldn’t have imagined, that isn’t going to work,” he told me.
This squid’s thought process is less alien to you than an artificial intelligence would be.
The second, subtler problem is that is that modern AI is very hard, even impossible, for humans to understand. Humans may write the initial algorithms, but the code will mutate as the machine learning system works its way by trial and error through vast datasets. Unlike traditional “deterministic” software, where the algorithms stay the same and a given input always produces the same output, machine learning AI reaches its conclusions along paths that humans did not create and cannot follow.
“Especially since the deep learning revolution happened in 2012, and you have all these algorithms, really complex algorithms, (there’s been) a lot of criticism…about how obscure, how opaque it is, how unreadable the results are,” Vila acknowledged. “But at the same time, I don’t think that has stopped the investment, (because) the performance is so, so much better than any other start of the art technique.”
Confidence in artificial intelligence won’t come from humans being able to audit the code line by line, Vila predicts, but from humans seeing it work consistently in real-world situations. That said, just as human eyes can be tricked by optical illusions, AI has quirks that enemies can exploit. (See our series on what we’re calling “Artificial Stupidity.”) So, Vila told me, “there needs to be an understanding across all levels, from the top commander all the way to the user, of what these algorithms can and cannot do, and even an understanding of how it can be fooled.”
Computer scientists and Pentagon leaders use the mythical centaur to describe their ideal of close collaboration between human and artificial intelligence.
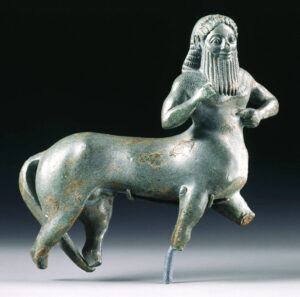
So a major goal for DARPA’s new program, and the focus for the $100 million in additional 2019 investment, is to develop a new generation of AI that can both function with smaller datasets and tell its users how and why it arrived at an answer. Walker called this the “third wave” of AI, after the first wave of deterministic rules-based systems and today’s second wave of statistical machine learning from big data.
DARPA wants to “give the machine the ability to reason in a contextual way about its environment,” Walker said. Only then, he said, can humans “move on from computer as a tool to computer as a partner.”
No comments:
Post a Comment