THE GIST: Although media headlines imply we are already living in a future where AI has infiltrated every aspect of society, this actually sets unrealistic expectations about what AI can really do for humanity. Governments around the world are racing to pledge support to AI initiatives, but they tend to understate the complexity around deploying advanced machine learning systems in the real world. This article reflects on the risks of “AI solutionism”: the increasingly popular belief that, given enough data, machine learning algorithms can solve all of humanity’s problems. There is no AI solution for everything. All solutions come at a cost and not everything that can be automated should be.
Hysteria about the future of artificial intelligence (AI) is everywhere. There is no shortage of sensationalist news about how AI could cure diseases, accelerate human innovation and improve human creativity. From the headlines alone, you might think we already live in a future where AI has infiltrated every aspect of society.
While it is undeniable that AI has opened up a wealth of promising opportunities, it has also led to the emergence of a mindset that can be best described as “AI solutionism”. This is the en vogue philosophy that, given enough data, machine learning algorithms can solve all of humanity’s problems.
But there’s a big problem with this idea. Instead of supporting AI progress, it actually jeopardises the value of machine intelligence by disregarding important AI safety principles and setting unrealistic expectations about what AI can really do for humanity.
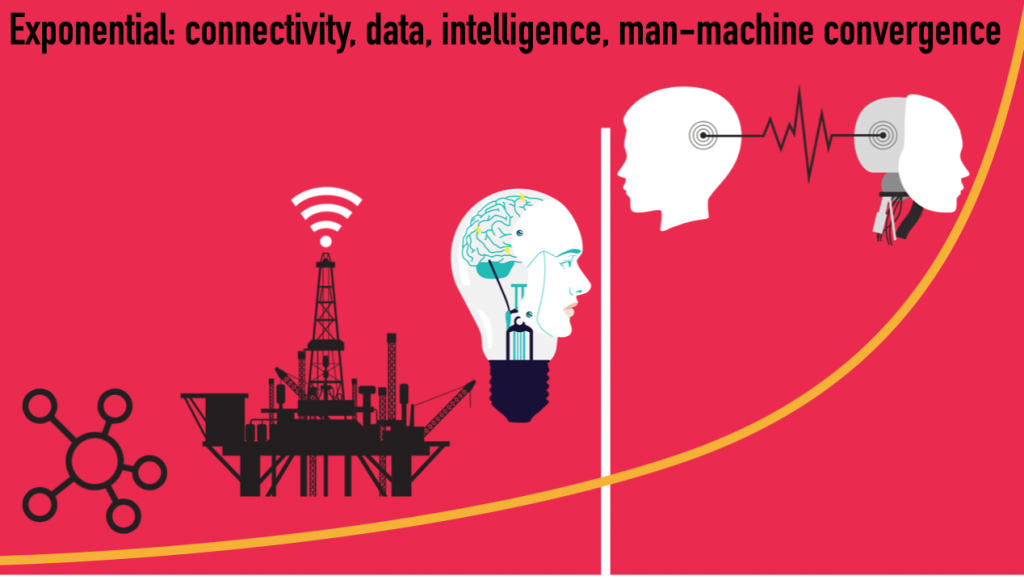
AI dreams and delusions
In only a few years, AI solutionism has spread from Silicon Valley’s technology evangelists to government officials and policymakers around the world. The pendulum has swung, away from the dystopian notion that AI will destroy humanity, towards the newly discovered utopian belief that our algorithmic saviour has arrived.
Around the world, we are now seeing governments pledge support to national AI initiatives and compete in a technological and rhetorical arms race to dominate the burgeoning machine learning sector. For example, the UK government has vowed to invest £300m in AI research, to position itself as a leader in the field. Enamoured with the transformative potential of AI, French president Emmanuel Macron has committed to turning France into a global AI hub. Meanwhile, the Chinese government is increasing its AI prowess with a national plan to create a Chinese AI industry worth $150 billion by 2030.
As prophetic science-fiction writer William Gibson once wrote, “the future is already here — it’s just not very evenly distributed.” And thus, many countries are hoping to dominate the Fourth Industrial Revolution. AI solutionism is on the rise, and it is here to stay.
“Governments should pause and take a deep breath when it comes to machine learning. Using AI simply for the sake of AI may not always be productive or useful.”
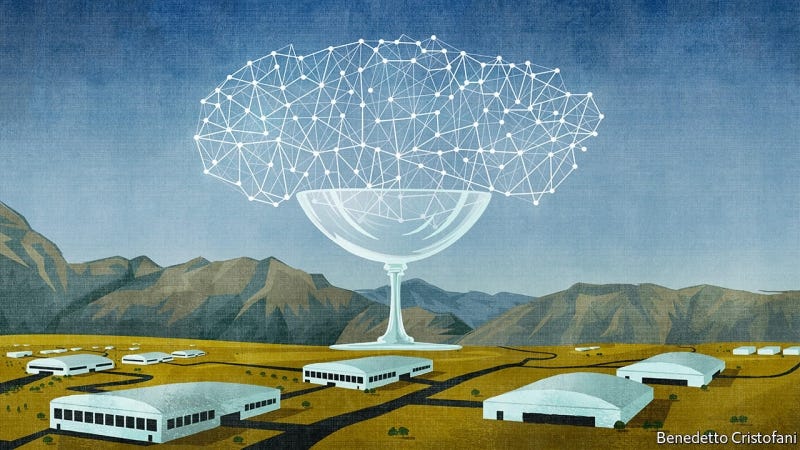
False promises or flawed premises?
While many political manifestos tout the positive transformative effects of the looming “AI revolution”, they tend to understate the complexity around deploying advanced machine learning systems in the real world. Naturally, there are limits to what AI can do, and these limits are linked to how machine learning technology actually works. To understand the reasons behind these misconceptions, let’s look at the basics.
One of the most promising varieties of AI technologies is neural networks. This form of machine learning is loosely modelled after the neuronal structure of the human brain, but on a much smaller scale. Many AI-based products use neural networks to infer patterns and rules from large volumes of data. But what many politicians do not understand is that simply adding a neural network to a problem will not automatically mean that you’ll find a solution.
“Simply adding a neural network to a democracy does not mean it will be instantly more inclusive, fair or personalised.”
If policymakers start deploying neural networks left right and centre, they should not assume that AI will instantly make our government institutions more agile or efficient. Simply adding a neural network to a democracy does not mean it will be instantaneously more inclusive, fair or personalised.
Consider, by way of analogy, transforming a physical shopping mall into a company such as Amazon. Simply adding a Facebook page or launching a website is not sufficient for the physical shopping mall to become a truly digital technology company. Something more is needed.
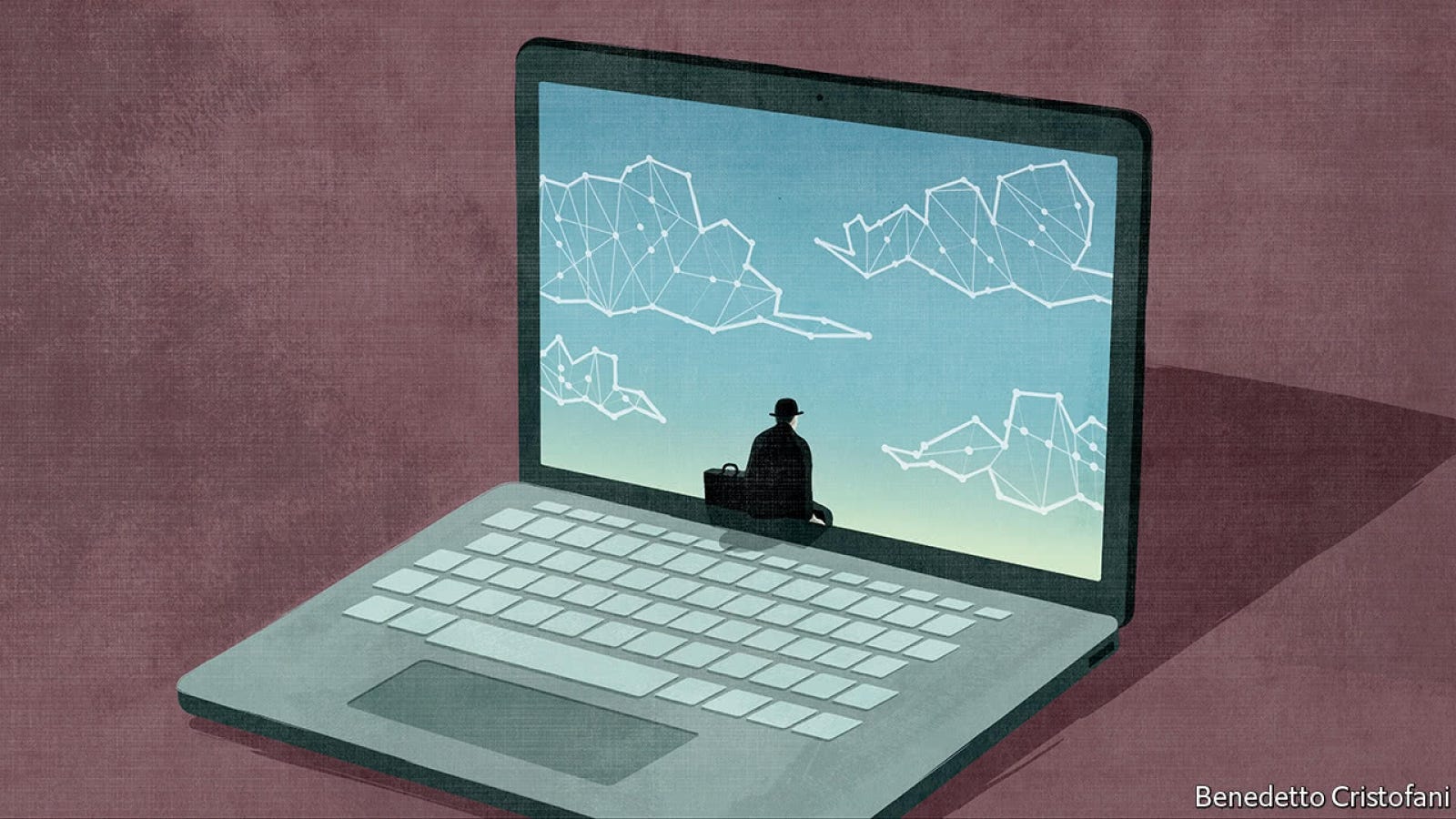
Challenging the data bureaucracy
AI systems need a lot of data to function. But the public sector typically does not have the appropriate data infrastructure to support advanced machine learning. Most of its data remain stored in offline archives. The few digitised sources of data that exist tend to be buried in bureaucracy. More often than not, data are spread across different government departments, each requiring special permission to be accessed. Above all, the public sector typically lacks the human talent with the right technological capabilities to reap the full benefits of machine intelligence.
Furthermore, one of the many difficulties in deploying machine learning systems is that AI is extremely susceptible to adversarial attacks. This means that a malicious AI can target another AI to force it to make wrong predictions or to behave in a certain way. Many researchers have warned against rolling out AI without appropriate security standards and defence mechanisms. Still, shockingly, AI security remains an often-overlooked topic in the political rhetoric of policymakers.
For these reasons, the media sensationalism around AI has attracted many critics. Stuart Russell, a professor of computer science at Berkeley, has long advocated a more realistic approach to neural networks, focusing on simple everyday applications of AI instead of the hypothetical robot takeover by a super-intelligent AI.
Similarly, MIT’s professor of robotics, Rodney Brooks, writes that “almost all innovations in robotics and AI take far, far, longer to be really widely deployed than people in the field and outside the field imagine”. Real progress is painful and slow. And in the case of AI, it requires a lot of data.
Making machines, not magic
If we are to reap the benefits and minimise the potential harms of AI, we must start thinking about how machine learning can be meaningfully applied to specific areas of government, business and society. This means we need to have a long-term multi-stakeholder discussion about AI ethics, and the distrust that many people still have towards machine learning.
Most importantly, we need to be aware of the limitations of AI, and of where humans still need to take the lead. Instead of painting an unrealistic picture of the superpowers afforded by AI robots, we should take a step back and separate AI’s actual technological capabilities from magic. Machine learning is neither magical fairy dust, nor the solution to everything.
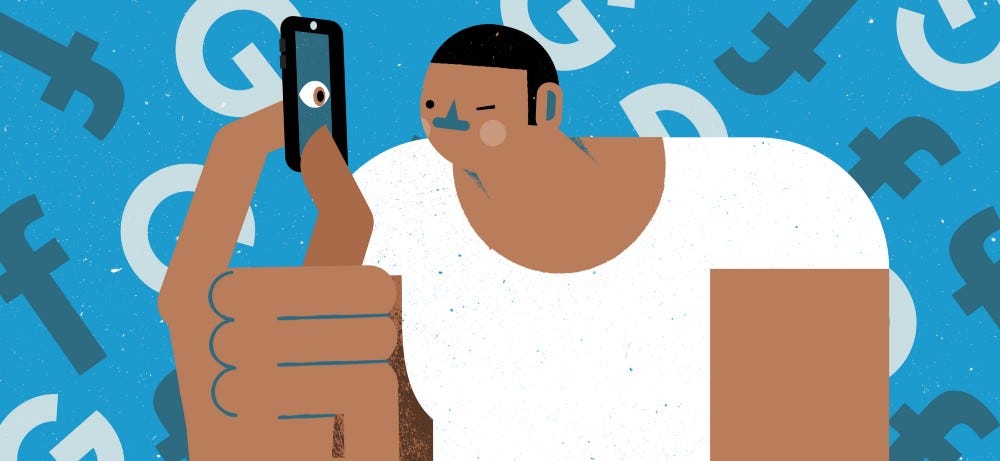
Even Facebook recently accepted that AI is not always the answer. For a long time, the social network believed that problems such as the spread of misinformation and hate speech could be algorithmically identified and stopped. But under recent pressure from legislators, the company quickly pledged to replace its algorithms with an army of more than 10,000 human reviewers.
The medical profession has also recognised that AI cannot be treated as panacea for all problems. The IBM Watson for Oncology programme was a piece of AI that was meant to help doctors treat cancer. Even though it was developed to deliver the best algorithmic recommendations, human experts found it difficult to trust the machine. As a result, the AI programme was abandoned in most hospitals where it was trialled, despite significant investments in the technology.
Similar problems arose in the legal domain, when algorithms were used in courts in America to sentence criminals. An opaque algorithm was used to calculate risk assessment scores and determine the likelihood that someone will commit another crime. The AI was designed to help judges make more data-centred decisions in court. However, the system was found to amplify structural racial discrimination, resulting in a major backlash from legal professionals and the general public.
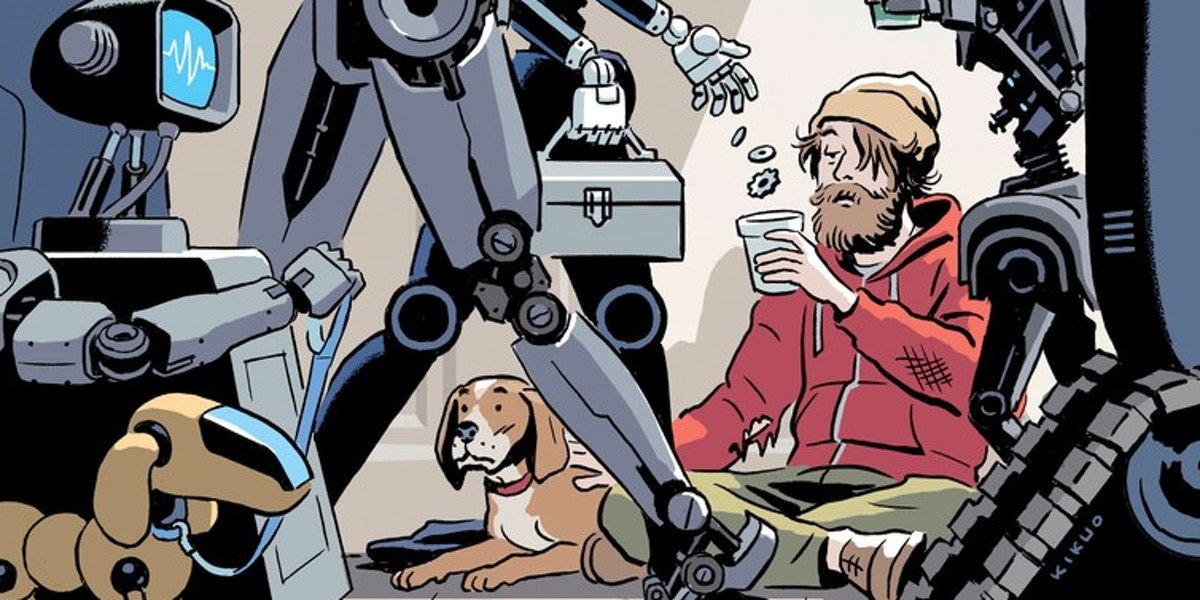
The law of AI instruments
These examples demonstrate that there is no AI solution for everything. Using AI simply for the sake of AI may not always be productive or useful. Not every problem is best addressed by applying machine intelligence.
In an almost prophetic treatise published in 1964, the American philosopher Abraham Kaplan described this tendency as the “law of the instrument”.
It was formulated as follows: “Give a small boy a hammer, and he will find that everything he encounters needs pounding”. Only this time, the mentality of Kaplan’s small boy is shared by influential world leaders, and the AI hammer is not only very powerful, but also very expensive.
Thus, this is the crucial lesson for everyone aiming to boost investments in expensive AI investments and national AI programmes: all solutions come with a cost, and not everything that can be automated should be.
We cannot afford to sit and wait until we reach general-level sentient AI in the distant future. Nor can we rely on narrow AI to solve all our problems for us today. We need to solve them ourselves today, while actively shaping new AI systems to help us in this monumental task.
About the author: Dr Vyacheslav Polonski is a researcher at the University of Oxford, studying complex social networks and collective behaviour. He holds a PhD in computational social science and has previously studied at Harvard, Oxford and LSE. He is actively involved in the World Economic Forum Expert Network and the WEF Global Shapers community, where he served as the Curator of the Oxford Hub. In 2018, Forbes Magazine featured his work and research on the Forbes 30 Under 30 list for Europe. He writes about the intersection of sociology, network science and technology.
Earlier versions of this article appeared in The Conversation, The Centre for Public Impact, Big Think and the World Economic Forum Agenda.
A huge thank you goes to the Ditchley conference on Machine Learning in 2017 for giving me a unique opportunity to refine my ideas and get early feedback from leading AI researchers, policy-makers and entrepreneurs in the field.
No comments:
Post a Comment