Geospatial imagery as well as facial recognition and other biometrics are driving the intelligence community’s research into artificial intelligence. Other intelligence activities, such as human language translation and event warning and forecasting, also stand to gain from advances being pursued in government, academic and industry research programs funded by the community’s research arm.
The Intelligence Advanced Research Projects Activity (IARPA) is working toward breakthroughs in artificial intelligence, or AI, through a number of research programs. All these AI programs tap expertise in government, industry or academia.
IARPA is one of the biggest financial backers of AI research, states its director, Jason Matheny, and imagery is the biggest growth area for intelligence AI. Imagery, including video, is the area of machine learning in which the community is most overwhelmed by data. The sheer quantity of imagery makes it impractical for humans to analyze all of it, so some form of automation is necessary. Imagery also is the area in which machine learning tools are most mature and most able to produce results quickly and accurately to enable deeper analysis. “Image recognition is probably the most mature application of machine learning, and the gains for national intelligence are enormous,” he states.
National intelligence is fundamentally about the ability to learn, to adapt and to achieve goals, Matheny notes. “The reason AI is needed in intelligence is that the world has scaled up in complexity, and there are scaling limits to human intelligence to make sense of that complexity,” he says.
This complexity has exceeded the point where even massive numbers of human analysts have sufficient brainpower to perform their mission, Matheny continues. Machine learning offers a way to bridge the gap between available resources and pressing needs. AI also allows the intelligence community to focus human brains and eyes where they are needed most.
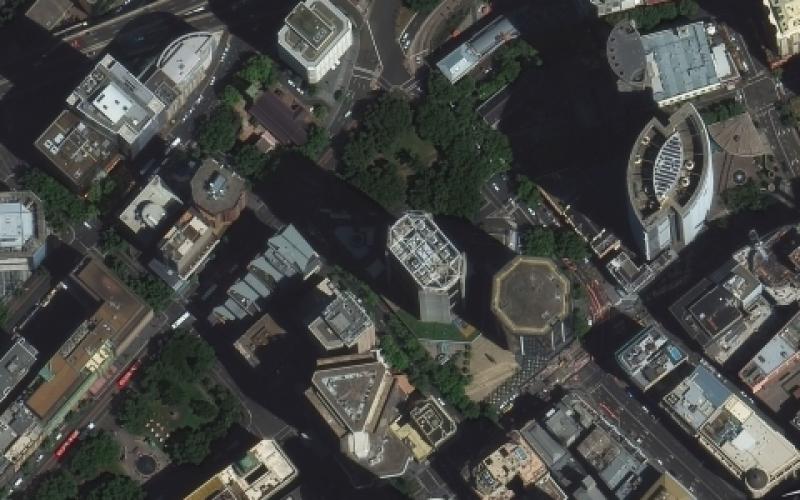
Its greatest application may be in areas that involve perceptual data, such as imagery, Matheny says. “Progress in machine learning on perceptual data has accelerated over the past several years,” he states. This is a result of the availability of large datasets, more affordable large-scale computing and better statistical techniques.
Although many on-the-shelf capabilities for processing imagery exist, more progress can be made in this area. “A lot can be done today to leverage existing tools and automate some aspects of intelligence so that an analyst could spend less time finding a tank and more time thinking about why the tank is there at all and what the tank might be doing tomorrow,” Matheny says. Today’s machine learning approaches can help find the tank, freeing up analysts to address the other two points—where machines cannot help.
Several IARPA programs focus on applying AI to national intelligence problems. The Finder program is developing a system that can determine where in the world a photograph was taken. It applies its encyclopedic knowledge of objects and geographic features, along with geolocation data. Another program, known as Creation of Operationally Realistic 3-D Environment (CORE3D), concentrates on building accurate 3-D models from overhead imagery. The program will apply data from multiple sources to generate automated models that capture the geometry and surface properties of objects on Earth more efficiently.
The Janus program is looking into facial recognition under realistic conditions, such as off-angle poses, bad lighting and poor resolution. It strives to fuse rich spatial, temporal and contextual information from multiple views captured by diverse sources. An effort known as ALADDIN, short for Automated Low-Level Analysis and Description of Diverse Intelligence Video, sifts through millions of online videos to find matches of areas of interest based on their contents. “These videos could be of a baseball game, a birthday party or someone providing instructions on how to make an IED [improvised explosive device],” Matheny illustrates. ALADDIN would be able to differentiate among them with a thorough scan.
The Deep Intermodal Video Analytics, or DIVA, program is working on activity detection and security. The idea is to develop technology that could automatically alert a security officer if a bag is being handed off or dropped, for example. This effort is related to the Janus, Finder and ALADDIN programs.
Another program, called Babel, looks for deep structure across and within human languages to learn how to transcribe any one of them with only a few hours of training data. Most transcription software today is derived from English, and it may not work as well on other languages. Babel seeks to remedy that shortcoming by developing a new approach to transcription. A related program, MATERIAL, short for Machine Translation for English Retrieval of Information in Any Language, applies machine learning to multilingual translation and knowledge discovery. It finds speech and text content in low-resource languages that is relevant to “domain-contextualized English queries,” according to an IARPA website about the program.
Several other programs go to the heart of AI applications such as alert systems. These systems combine data from different streams to provide early warning of important events, such as political instability, military mobilization, disease outbreaks or cyber attacks. During a disease outbreak, for example, use of disease-related keywords in social media or web search queries may suddenly increase, Matheny notes. Mobile device geolocation data might show anomalies such as an unusually high number of people staying at home from work.
Machine learning can identify these types of indicators, Matheny points out. IARPA’s Open Source Indicators (OSI), Cyber-attack Automated Unconventional Sensor Environment (CAUSE) and Mercury programs look for indicators of future events in data.
Still other programs focus on developing the next generation of machine learning technologies. The Machine Intelligence from Cortical Networks (MICrONS) program seeks to understand how the brain computes, which would help IARPA produce more efficient machine learning algorithms, Matheny suggests. He notes that the most popular machine learning method, deep neural nets, is based on a 1950s model on how the brain computes. “We’ve learned a bit since the 1950s, so MICrONS is aimed at leveraging a more realistic model of computation in the brain,” he posits.
These programs are only one part of IARPA’s AI picture. The agency also funds prizes in machine learning. The advantage of this approach is that competitors can skip the proposal and just submit the solution. If a competitor’s solution tops all others, then it wins money. IARPA recently completed a prize challenge on 3-D mapping for imagery, and it launched a prize challenge in July called Functional Map of the World. This will help infer the functions of a building just by looking at its structure.
Most of these IARPA programs aim to benefit from open source information in cyberspace. IARPA also is pursuing AI applications to assist with sorting through information collected by traditional intelligence means. One recently completed program titled Knowledge, Discovery and Dissemination, or KDD, helps align data from different sources. This data could come from analytic or field reports, Matheny allows. KDD generated many components that already are being used to align data, he adds.
With its full roster of programs, IARPA faces some hurdles in developing AI for intelligence. Matheny allows that one involves finding appropriate datasets for training, testing and benchmarking. He admits that IARPA spends a large amount of money on data that can be released to researchers. This must be either existing unclassified data or something resembling classified data, which IARPA must cobble together from unclassified sources. These datasets would be used to train or test systems that are deployed against classified data.
Full disclosure: SIGNAL Magazine provided its database of articles going back more than a decade free of charge to the Office of the Director of National Intelligence’s (ODNI’s) Xpress Challenge. This allowed contestants to exercise their entries in a controlled dataset that featured many topics of importance to intelligence searches.
Another issue for IARPA is explainability or transparency. IARPA requires that systems generating warnings or forecasts explain to human users why they produced the results they offered. Matheny describes the importance of this transparency by noting that intelligence analysts are unlikely to trust a system unless they understand how its results are achieved.
“One reason this is challenging is that in deep learning—a popular form of machine learning—the methods are fairly opaque to human inspection,” Matheny points out. “To explain the results in natural language often requires a large amount of work.” He adds that the Defense Advanced Research Projects Agency (DARPA) also is working on a program for explainable AI. “If you don’t bake in explainability from the start, how do you sort of retrofit your system to make it explainable?” he poses.
A third AI challenge is causality, which involves event analysis. Present-day machine learning and statistical methods are good at identifying correlations in data, Matheny explains. But they are not good at determining cause and effect, which is important to decision makers. AI must be able to separate causality from coincidence.
Another challenge is robustness against spoofing or “adversarial inputs,” Matheny says. Concerns are growing about how various AI systems could be spoofed through fairly simple data inputs via hacks. “It has become a parlor trick to show how an image-recognition system could be fooled or confused if pixels are misplaced here or there,” he allows. A picture of a tank could be misread as a picture of a school bus, even though the human eye easily could discern the difference.
“There is a lot of mischief that could be done with those sorts of techniques,” he reveals. Denial and deception has emigrated from the battlespace to the digital domain, and IARPA has assigned a high degree of importance to finding ways of protecting against this kind of spoofing.
IARPA also is interested in coordinating its AI research strategy with other organizations, so it works closely with groups such as DARPA, the National Science Foundation and the National Institute of Standards and Technology. There is broad recognition across the government that efforts to develop and adopt AI for the public good will not succeed without deeper public-private partnerships, Matheny observes.
IARPA’s business model is to fund organizations in academia and industry that already are on the cutting edge of their research fields, he notes. Open broad agency announcements solicit proposals related to any element of intelligence work. Matheny describes the process for submitting ideas as informal.
“What we want most are the ideas that we wouldn’t have thought of ourselves,” he says. “We don’t just want industry or academia to parrot back to the government what the government is asking for. We want new breakthrough ideas that we couldn’t have come up with ourselves—and we might not even be asking the questions to solicit [the ideas].”
A priority is to ensure that the machine learning systems industry develops and embeds in technologies have some level of security against adversaries, Matheny says. Systems that will be used for geospatial or signals intelligence should at least be tested against known cyber attacks, particularly those designed to confuse classifiers, a type of AI application, he adds. Industry must begin addressing this area in its own internal testing processes.
Within the government, the CIA’s venture capital firm, In-Q-Tel, has its own section focusing on AI. Matheny explains that IARPA works with In-Q-Tel to understand which AI technologies are commercially ready. Sometimes, the end of an IARPA AI research program leads to a startup, and IARPA will collaborate with In-Q-Tel to determine whether the firm should receive private funding and how much. Dialogue between IARPA and In-Q-Tel also helps the research organization avoid duplicating industry projects, Matheny relates.
AI and machine learning implications for intelligence will be the focus of a panel discussion moderated by Jason Matheny, IARPA director, at the Intelligence and National Security Summit on Wednesday, September 6, in Washington, D.C. The summit is September 6-7.
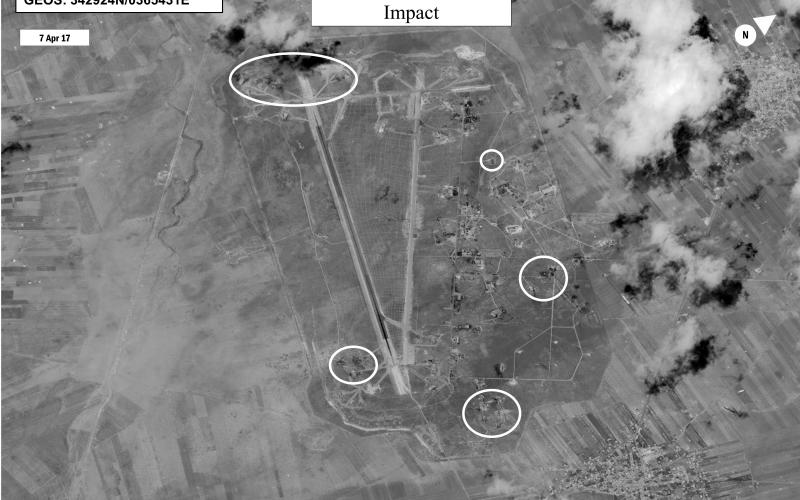
Imagery of U.S. missile strikes on Shayrat airfield, Syria, shows the damage after the onslaught. Surveillance and reconnaissance imagery risks tampering by malware injected into AI processing software, requiring built-in security before a machine learning system is deployed. Photo credit: U.S. Navy/DigitalGlobe
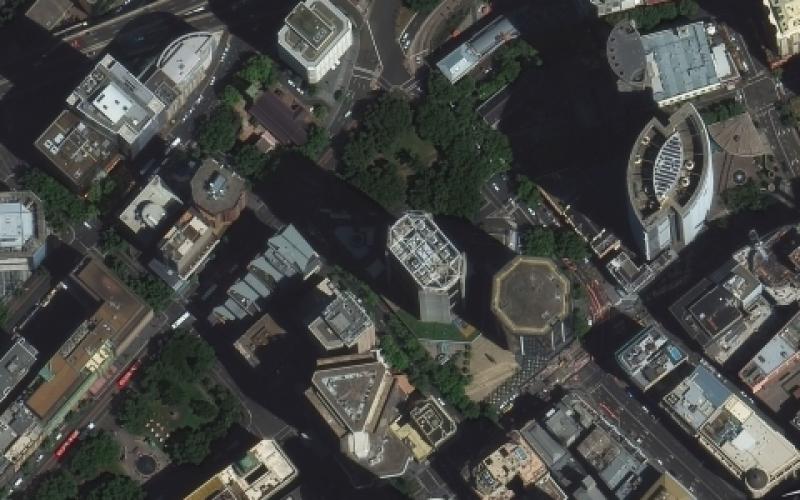
A DigitalGlobe WorldView-3 satellite image of Sydney shows the variety of buildings dotting the landscape. Among the artificial intelligence (AI) research sponsored by the Intelligence Advanced Research Projects Activity (IARPA) is an AI program designed to determine the functions of buildings just from looking at overhead imagery.
No comments:
Post a Comment